Probability distribution fitting
Probability distribution fitting or simply distribution fitting is the fitting of a probability distribution to a series of data concerning the repeated measurement of a variable phenomenon.
The aim of distribution fitting is to predict the probability or to forecast the frequency of occurrence of the magnitude of the phenomenon in a certain interval.
There are many probability distributions (see list of probability distributions) of which some can be fitted more closely to the observed frequency of the data than others, depending on the characteristics of the phenomenon and of the distribution. The distribution giving a close fit is supposed to lead to good predictions.
In distribution fitting, therefore, one needs to select a distribution that suits the data well.
Distribution selection

The selection of the appropriate distribution depends on the presence or absence of symmetry of the data set with respect to the mean value.
Symmetrical distributions
When the data are symmetrically distributed around the mean while the frequency of occurrence of data farther away from the mean diminishes, one may for example select for example the normal distribution, the logistic distribution, or the Student's t-distribution. The first two are very similar, while the last, with one degree of freedom, has "heavier tails" meaning that the values farther away from the mean occur relatively more often (i.e. the kurtosis is higher). The Cauchy distribution is also symmetrical.
Skew distributions to the right
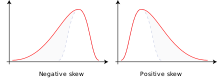
When the larger values tend to be farther away from the mean than the smaller values, one has a skew distribution to the right (i.e. there is positive skewness), one may for example select the log-normal distribution (i.e. the log values of the data are normally distributed), the log-logistic distribution (i.e. the log values of the data follow a logistic distribution), the Gumbel distribution, the exponential distribution, the Pareto distribution, the Weibull distribution, or the Fréchet distribution. These distributions are generally bounded to the left.
Skew distributions to the left
When the smaller values tend to be farther away from the mean than the larger values, one has a skew distribution to the left (i.e. there is negative skewness), one may for example select the square-normal distribution (i.e. the normal distribution applied to the square of the data values), the inverted (mirrored) Gumbel distribution, or the Gompertz distribution. These distributions are generally bounded to the right.
Fitting techniques
The following techniques of distribution fitting exist:[1]
- Parametric methods, by which the parameters of the distribution are calculated from the data series.[2] The parametric methods are:
- method of moments
- method of L-moments[3]
- Maximum likelihood method[4]
For example, the parameter μ (the expectation) can be estimated by the mean of the data and the parameter σ 2 (the variance) can be estimated from the standard deviation of the data. The mean is found as m = Σ(X) / n, where X is the data value and n the number of data, while the standard deviation is calculated as s =√{Σ(X-m)2 / (n-1)}. With these parameters many distributions, e.g. the normal distribution, are completely defined.

- Regression method, using a transformation of the cumulative distribution function so that a linear relation is found between the cumulative probability and the values of the data, which may also need to be transformed, depending on the selected probability distribution. In this method the cumulative probability needs to be estimated by the plotting position.
For example, the cumulative Gumbel distribution can be linearized to Y = aX+b, where X is the data variable and Y = -ln(-lnP), with P being the cumulative probability, i.e. the probability that the data value is less than X. Thus, using the plotting position for P, one finds the parameters a and b from a linear regression of Y on X, and the Gumbel distribution is fully defined.
Prediction uncertainty
Predictions of occurrence based on fitted probability distributions are subject to uncertainty, which arises from the following conditions:
- The true probability distribution of events may deviate from the fitted distribution, as the observed data series may not be totally representative of the real probability of occurrence of the phenomenon due to random error
- The occurrence of events in another situation or in the future may deviate from the fitted distribution as the this occurrence can also be subject to random error
- A change of environmental conditions may cause a change in the probability of occurrence of the phenomenon
Hence, in prediction one needs to take uncertainty into account.
An estimate of the uncertainty in the first case can be obtained with the binomial distribution using for example the probability of exceedance Pe (i.e. the chance that the event is larger than a reference value X), and the probability of non-exceedance Pn (i.e. the chance that the event is smaller than or equal to the reference value X). Another option is using the probability of occurrence inside a certain range Pi (i.e. the chance that the event is in a range between a lower limit L and upper limit U) or outside that range (Po). In these cases, there are only two possibilities: either there is exceedance or there is non-exceedance, respectively the event either occurs inside the range or it falls outside it. This makes that the binomial distribution is applicable.
An example of the application of the binomial distribution to obtain a confidence interval of the prediction is given in frequency analysis. Such an interval also estimates the risk of failure, i.e. the chance that the predicted event still remains outside the confidence interval.
References
- ^ Frequency and Regression Analysis. Chapter 6 in: H.P.Ritzema (ed., 1994), Drainage Principles and Applications, Publ. 16, pp. 175–224, International Institute for Land Reclamation and Improvement (ILRI), Wageningen, The Netherlands. ISBN 9070754339. Free download from the from webpage [1] under nr. 12, or directly as PDF : [2]
- ^ H. Cramér, "Mathematical methods of statistics" , Princeton Univ. Press (1946)
- ^ Hosking, J.R.M. (1990). "L-moments: analysis and estimation of distributions using linear combinations of order statistics". Journal of the Royal Statistical Society, Series B. 52: 105–124. JSTOR 2345653.
- ^ Aldrich, John (1997). "R. A. Fisher and the making of maximum likelihood 1912–1922". Statistical Science. 12 (3): 162–176. doi:10.1214/ss/1030037906. MR 1617519.