Smoothing
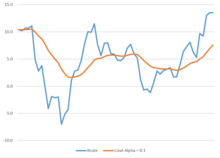
In statistics and image processing, to smooth a data set is to create an approximating function that attempts to capture important patterns in the data, while leaving out noise or other fine-scale structures/rapid phenomena. In smoothing, the data points of a signal are modified so individual points higher than the adjacent points (presumably because of noise) are reduced, and points that are lower than the adjacent points are increased leading to a smoother signal. Smoothing may be used in two important ways that can aid in data analysis (1) by being able to extract more information from the data as long as the assumption of smoothing is reasonable and (2) by being able to provide analyses that are both flexible and robust.[1] Many different algorithms are used in smoothing.
Smoothing may be distinguished from the related and partially overlapping concept of curve fitting in the following ways:
- curve fitting often involves the use of an explicit function form for the result, whereas the immediate results from smoothing are the "smoothed" values with no later use made of a functional form if there is one;
- the aim of smoothing is to give a general idea of relatively slow changes of value with little attention paid to the close matching of data values, while curve fitting concentrates on achieving as close a match as possible.
- smoothing methods often have an associated tuning parameter which is used to control the extent of smoothing. Curve fitting will adjust any number of parameters of the function to obtain the 'best' fit.
Linear smoothers
[edit]In the case that the smoothed values can be written as a linear transformation of the observed values, the smoothing operation is known as a linear smoother; the matrix representing the transformation is known as a smoother matrix or hat matrix.[citation needed]
The operation of applying such a matrix transformation is called convolution. Thus the matrix is also called convolution matrix or a convolution kernel. In the case of simple series of data points (rather than a multi-dimensional image), the convolution kernel is a one-dimensional vector.
Algorithms
[edit]One of the most common algorithms is the "moving average", often used to try to capture important trends in repeated statistical surveys. In image processing and computer vision, smoothing ideas are used in scale space representations. The simplest smoothing algorithm is the "rectangular" or "unweighted sliding-average smooth". This method replaces each point in the signal with the average of "m" adjacent points, where "m" is a positive integer called the "smooth width". Usually m is an odd number. The triangular smooth is like the rectangular smooth except that it implements a weighted smoothing function.[2]
Some specific smoothing and filter types, with their respective uses, pros and cons are:
Algorithm | Overview and uses | Pros | Cons |
---|---|---|---|
Additive smoothing | used to smooth categorical data. | ||
Butterworth filter | Slower roll-off than a Chebyshev Type I/Type II filter or an elliptic filter |
|
|
Chebyshev filter | Has a steeper roll-off and more passband ripple (type I) or stopband ripple (type II) than Butterworth filters. |
|
|
Digital filter | Used on a sampled, discrete-time signal to reduce or enhance certain aspects of that signal | ||
Elliptic filter | |||
Exponential smoothing |
|
||
Kalman filter |
|
Estimates of unknown variables it produces tend to be more accurate than those based on a single measurement alone | |
Kernel smoother | The estimated function is smooth, and the level of smoothness is set by a single parameter. | ||
Kolmogorov–Zurbenko filter |
|
|
|
Laplacian smoothing | algorithm to smooth a polygonal mesh.[4][5] | ||
Local regression also known as "loess" or "lowess" | a generalization of moving average and polynomial regression. |
|
|
Low-pass filter |
|
||
Moving average |
|
|
|
Ramer–Douglas–Peucker algorithm | decimates a curve composed of line segments to a similar curve with fewer points. | ||
Savitzky–Golay smoothing filter |
|
||
Smoothing spline | |||
Stretched grid method |
|
See also
[edit]- Convolution
- Curve fitting
- Discretization
- Edge preserving smoothing
- Filtering (signal processing)
- Graph cuts in computer vision
- Numerical smoothing and differentiation
- Scale space
- Scatterplot smoothing
- Smoothing spline
- Smoothness
- Statistical signal processing
- Subdivision surface, used in computer graphics
- Window function
References
[edit]- ^ Simonoff, Jeffrey S. (1998) Smoothing Methods in Statistics, 2nd edition. Springer ISBN 978-0387947167 [page needed]
- ^ O'Haver, T. (January 2012). "Smoothing". terpconnect.umd.edu.
- ^ a b Easton, V. J.; & McColl, J. H. (1997)"Time series", STEPS Statistics Glossary
- ^ Herrmann, Leonard R. (1976), "Laplacian-isoparametric grid generation scheme", Journal of the Engineering Mechanics Division, 102 (5): 749–756, doi:10.1061/JMCEA3.0002158.
- ^
Sorkine, O., Cohen-Or, D., Lipman, Y., Alexa, M., Rössl, C., Seidel, H.-P. (2004). "Laplacian Surface Editing". Proceedings of the 2004 Eurographics/ACM SIGGRAPH Symposium on Geometry Processing. SGP '04. Nice, France: ACM. pp. 175–184. doi:10.1145/1057432.1057456. ISBN 3-905673-13-4. S2CID 1980978.
{{cite book}}
: CS1 maint: multiple names: authors list (link)
Further reading
[edit]- Hastie, T.J. and Tibshirani, R.J. (1990), Generalized Additive Models, New York: Chapman and Hall.