Aggregate function
In database management, an aggregate function or aggregation function is a function where multiple values are processed together to form a single summary statistic.
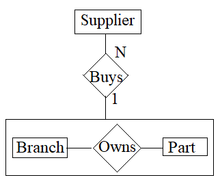
Common aggregate functions include:
Others include:
- Nanmean (mean ignoring NaN values, also known as "nil" or "null")
- Stddev
Formally, an aggregate function takes as input a set, a multiset (bag), or a list from some input domain I and outputs an element of an output domain O.[1] The input and output domains may be the same, such as for SUM
, or may be different, such as for COUNT
.
Aggregate functions occur commonly in numerous programming languages, in spreadsheets, and in relational algebra.
The listagg
function, as defined in the SQL:2016 standard[2]
aggregates data from multiple rows into a single concatenated string.
In the entity relationship diagram, aggregation is represented as seen in Figure 1 with a rectangle around the relationship and its entities to indicate that it is being treated as an aggregate entity.[3]
Decomposable aggregate functions[edit]
Aggregate functions present a bottleneck, because they potentially require having all input values at once. In distributed computing, it is desirable to divide such computations into smaller pieces, and distribute the work, usually computing in parallel, via a divide and conquer algorithm.
Some aggregate functions can be computed by computing the aggregate for subsets, and then aggregating these aggregates; examples include COUNT
, MAX
, MIN
, and SUM
. In other cases the aggregate can be computed by computing auxiliary numbers for subsets, aggregating these auxiliary numbers, and finally computing the overall number at the end; examples include AVERAGE
(tracking sum and count, dividing at the end) and RANGE
(tracking max and min, subtracting at the end). In other cases the aggregate cannot be computed without analyzing the entire set at once, though in some cases approximations can be distributed; examples include DISTINCT COUNT
(Count-distinct problem), MEDIAN
, and MODE
.
Such functions are called decomposable aggregation functions[4] or decomposable aggregate functions. The simplest may be referred to as self-decomposable aggregation functions, which are defined as those functions f such that there is a merge operator such that
where is the union of multisets (see monoid homomorphism).
For example, SUM
:
- , for a singleton;
- , meaning that merge is simply addition.
COUNT
:
- ,
- .
MAX
:
- ,
- .
MIN
:
- ,[2]
- .
Note that self-decomposable aggregation functions can be combined (formally, taking the product) by applying them separately, so for instance one can compute both the SUM
and COUNT
at the same time, by tracking two numbers.
More generally, one can define a decomposable aggregation function f as one that can be expressed as the composition of a final function g and a self-decomposable aggregation function h, . For example, AVERAGE
=SUM
/COUNT
and RANGE
=MAX
−MIN
.
In the MapReduce framework, these steps are known as InitialReduce (value on individual record/singleton set), Combine (binary merge on two aggregations), and FinalReduce (final function on auxiliary values),[5] and moving decomposable aggregation before the Shuffle phase is known as an InitialReduce step,[6]
Decomposable aggregation functions are important in online analytical processing (OLAP), as they allow aggregation queries to be computed on the pre-computed results in the OLAP cube, rather than on the base data.[7] For example, it is easy to support COUNT
, MAX
, MIN
, and SUM
in OLAP, since these can be computed for each cell of the OLAP cube and then summarized ("rolled up"), but it is difficult to support MEDIAN
, as that must be computed for every view separately.
Other decomposable aggregate functions[edit]
In order to calculate the average and standard deviation from aggregate data, it is necessary to have available for each group: the total of values (Σxi = SUM(x)), the number of values (N=COUNT(x)) and the total of squares of the values (Σxi2=SUM(x2)) of each groups.[8]
AVG
:
SUM(x2)
:
The sum of squares of the values is important in order to calculate the Standard Deviation of groupsSTDDEV
:For a finite population with equal probabilities at all points, we have[9][circular reference]
This means that the standard deviation is equal to the square root of the difference between the average of the squares of the values and the square of the average value.
See also[edit]
- Cross-tabulation a.k.a. Contingency table
- Data drilling
- Data mining
- Data processing
- Extract, transform, load
- Fold (higher-order function)
- Group by (SQL), SQL clause
- OLAP cube
- Online analytical processing
- Pivot table
- Relational algebra
- Utility functions on indivisible goods#Aggregates of utility functions
- XML for Analysis
- AggregateIQ
- MapReduce
References[edit]
- ^ Jesus, Baquero & Almeida 2011, 2 Problem Definition, pp. 3.
- ^ a b Winand, Markus (2017-05-15). "Big News in Databases: New SQL Standard, Cloud Wars, and ACIDRain (Spring 2017)". DZone. Archived from the original on 2017-05-27. Retrieved 2017-06-10.
In December 2016, ISO released a new version of the SQL standard. It introduces new features such as row pattern matching, listagg, date and time formatting, and JSON support.
- ^ Elmasri, Ramez (2016). Fundamentals of database systems. Sham Navathe (Seventh ed.). Hoboken, NJ. p. 133. ISBN 978-0-13-397077-7. OCLC 913842106.
{{cite book}}
: CS1 maint: location missing publisher (link) - ^ Jesus, Baquero & Almeida 2011, 2.1 Decomposable functions, pp. 3–4.
- ^ Yu, Gunda & Isard 2009, 2. Distributed Aggregation, pp. 2–4.
- ^ Yu, Gunda & Isard 2009, 2. Distributed Aggregation, p. 1.
- ^ Zhang 2017, p. 1.
- ^ Ing. Óscar Bonilla, MBA
- ^ Standard deviation#Identities and mathematical properties
Literature[edit]
- Grabisch, Michel; Marichal, Jean-Luc; Mesiar, Radko; Pap, Endre (2009). Aggregation functions. Encyclopedia of Mathematics and its Applications. Vol. 127. Cambridge: Cambridge University Press. ISBN 978-0-521-51926-7. Zbl 1196.00002.
- Oracle Aggregate Functions: MAX, MIN, COUNT, SUM, AVG Examples
- Yu, Yuan; Gunda, Pradeep Kumar; Isard, Michael (2009). Distributed aggregation for data-parallel computing: interfaces and implementations. ACM SIGOPS 22nd symposium on Operating systems principles. ACM. pp. 247–260. doi:10.1145/1629575.1629600.
- Jesus, Paulo; Baquero, Carlos; Almeida, Paulo Sérgio (2011). "A Survey of Distributed Data Aggregation Algorithms". arXiv:1110.0725 [cs.DC].
- Zhang, Chao (2017). Symmetric and Asymmetric Aggregate Function in Massively Parallel Computing (Technical report).