Data editing
Data editing is defined as the process involving the review and adjustment of collected survey data.[1] Data editing helps define guidelines that will reduce potential bias and ensure consistent estimates leading to a clear analysis of the data set by correct inconsistent data using the methods later in this article.[2] The purpose is to control the quality of the collected data.[3] Data editing can be performed manually, with the assistance of a computer or a combination of both.[4]
Editing methods
[edit]Editing methods refer to a range of procedures and processes used for detecting and handling errors in data. Data editing is used with the goal to improve the quality of statistical data produced. These modifications can greatly improve the quality of analytics created by aiming to detect and correct errors. Examples of different techniques to data editing such as micro-editing, macro-editing, selective editing, or the different tools used to achieve data editings such as graphical editing and interactive editing.
Interactive editing
[edit]The term interactive editing is commonly used for modern computer-assisted manual editing. Most interactive data editing tools applied at National Statistical Institutes (NSIs) allow one to check the specified edits during or after data entry, and if necessary to correct erroneous data immediately. Several approaches can be followed to correct erroneous data:
- Re-contact the respondent
- Compare the respondent's data to his data from the previous year
- Compare the respondent's data to data from similar respondents
- Use the subject matter knowledge of the human editor
Interactive editing is a standard way to edit data. It can be used to edit both categorical and continuous data.[5] Interactive editing reduces the time frame needed to complete the cyclical process of review and adjustment.[6] Interactive editing also requires an understanding of the data set and the possible results that would come from an analysis of the data.
Selective editing
[edit]Selective editing is an umbrella term for several methods to identify the influential errors, [note 1] and outliers.[note 2] Selective editing techniques aim to apply interactive editing to a well-chosen subset of the records, such that the limited time and resources available for interactive editing are allocated to those records where it has the most effect on the quality of the final estimates of published figures. In selective editing, data is split into two streams:
- The critical stream
- The non-critical stream
The critical stream consists of records that are more likely to contain influential errors. These critical records are edited in a traditional interactive manner. The records in the non-critical stream which are unlikely to contain influential errors are not edited in a computer-assisted manner.[7]
Data editing techniques
[edit]Data editing can be accomplished in many ways and primarily depends on the data set that is being explored.[8]
Validity and completeness of data
[edit]The validity of a data set depends on the completeness of the responses provided by the respondents. One method of data editing is to ensure that all responses are complete in fields that require a numerical or non-numerical answer. See the example below.

Duplicate data entry
[edit]Verifying that the data is unique is an important aspect of data editing to ensure that all data provided was only entered once. This reduces the possibility for repeated data that could skew analytics reporting. See the example below.

Outliers
[edit]It is common to find outliers in data sets, which as described before are values that do not fit a model of data well. These extreme values can be found based on the distribution of data points from previous data series or parallel data series for the same data set. The values can be considered erroneous and require further analysis for checking and determining the validity of the response. See the example below.

Logical inconsistencies
[edit]Logical consistency is the presence of logical relationships and interdependence between the variables. This editing requires a certain understanding around the dataset and the ability to identify errors in data based on previous reports or information. This type of data editing is used to account for the differences between data fields or variables. See the example below.
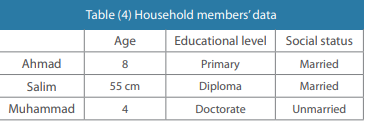
Macro editing
[edit]There are two methods of macro editing:[7]
Aggregation method
[edit]This method is followed in almost every statistical agency before publication: verifying whether figures to be published seems plausible. This is accomplished by comparing quantities in publication tables with the same quantities in previous publications. If an unusual value is observed, a micro-editing procedure is applied to the individual records and fields contributing to the suspicious quantity.[6]
Distribution method
[edit]Data available is used to characterize the distribution of the variables. Then all individual values are compared with the distribution. Records containing values that could be considered uncommon (given the distribution) are candidates for further inspection and possibly for editing.[9]
Automatic editing
[edit]In automatic editing records are edited by a computer without human intervention.[10] Prior knowledge on the values of a single variable or a combination of variables can be formulated as a set of edit rules which specify or constrain the admissible values
Determinants of data editing
[edit]Data editing has its limitations with the capacity and resources of any given study. These determinants can have a positive or negative impact on the post-analysis of the data set. Below are several determinants of data editing.[8]
Available resources: [8]
- Time allocated to the project
- Money and budget constraints
Available Software:[8]
- Tools used to analyze the data
- Tools available to identify errors in the data set
- Immediate availability of software depending on the objectives and goals of the data
Data Source: [8]
- Limitations of respondents to answer according to expectations
- Missing information from respondents that are not readily available
- Follow-ups are difficult to maintain in large data pools
Coordination of Data Editing Procedure: [8]
- Subjective views on the data set
- Disagreements between the overall objectives of the data
- Methods used to handle data editing
See also
[edit]- Data cleansing
- Data pre-processing
- Data wrangling
- Iterative proportional fitting
- Triangulation (social science)
Notes
[edit]References
[edit]- ^ Ferguson, Dania P. "AN INTRODUCTION TO THE DATA EDITING PROCESS" (PDF). unece.org/.
- ^ "National Center for Education Statistics (NCES) Home Page, part of the U.S. Department of Education". nces.ed.gov. Retrieved 2020-12-06.
- ^ "UNECE".
- ^ "Stat¡stics: Power from Data! Data editing". www150.statcan.gc.ca.
- ^ Waal, Ton de et al. "Handbook of Statistical Data Editing and Imputation". Wiley publication, 2011, p.15.
- ^ a b "UNECE Homepage". www.unece.org.
- ^ a b Waal, Ton de et al. "Handbook of Statistical Data Editing and Imputation". Wiley publication, 2011, p.16.
- ^ a b c d e f SCAD. "SCAD". SCAD. Retrieved 2020-12-07.
- ^ Bethlehem, J. "Applied Survey Methods A Statistical Perspective ". Wiley publication, 2009, p.205.
- ^ Waal, Ton de et al. "Handbook of Statistical Data Editing and Imputation". Wiley publication