Meta-analysis: Difference between revisions
Citation bot (talk | contribs) Alter: url. URLs might have been anonymized. Add: s2cid, pmid, authors 1-1. Removed parameters. Some additions/deletions were parameter name changes. | Use this bot. Report bugs. | Suggested by AManWithNoPlan | #UCB_webform 492/783 |
consistent citation formatting; templated cites |
||
Line 17: | Line 17: | ||
Not only can meta-analyses provide an estimate of the unknown effect size, it also has the capacity to contrast results from different studies and identify patterns among study results, sources of disagreement among those results, or other interesting relationships that may come to light with multiple studies.<ref>Greenland S, O' Rourke K: Meta-Analysis. Page 652 in Modern Epidemiology, 3rd ed. Edited by Rothman KJ, Greenland S, Lash T. Lippincott Williams and Wilkins; 2008.</ref> |
Not only can meta-analyses provide an estimate of the unknown effect size, it also has the capacity to contrast results from different studies and identify patterns among study results, sources of disagreement among those results, or other interesting relationships that may come to light with multiple studies.<ref>Greenland S, O' Rourke K: Meta-Analysis. Page 652 in Modern Epidemiology, 3rd ed. Edited by Rothman KJ, Greenland S, Lash T. Lippincott Williams and Wilkins; 2008.</ref> |
||
However, there are some methodological problems with meta-analysis. If individual studies are systematically biased due to questionable research practices (e.g., p-hacking, data peeking, dropping studies) or the publication bias at the journal level, the meta-analytic estimate of the overall treatment effect may not reflect the actual efficacy of a treatment<ref>{{Cite book| |
However, there are some methodological problems with meta-analysis. If individual studies are systematically biased due to questionable research practices (e.g., p-hacking, data peeking, dropping studies) or the publication bias at the journal level, the meta-analytic estimate of the overall treatment effect may not reflect the actual efficacy of a treatment<ref>{{Cite book| vauthors = Tabery J |url=https://books.google.com/books?id=aeCOAwAAQBAJ&pg=PR9|title=Beyond Versus: The Struggle to Understand the Interaction of Nature and Nurture|date=2014-05-09|publisher=MIT Press|isbn=978-0-262-02737-3|language=en}}</ref><ref name="Hunter&Schmidt1990" />. Meta-analysis has also been criticized for averaging differences among heterogeneous studies because these differences could potentially inform clinical decisions<ref>{{cite journal | vauthors = Maziarz M | title = Is meta-analysis of RCTs assessing the efficacy of interventions a reliable source of evidence for therapeutic decisions? | journal = Studies in History and Philosophy of Science | volume = 91 | pages = 159–167 | date = December 2021 | pmid = 34922183 | doi = 10.1016/j.shpsa.2021.11.007 | s2cid = 245241150 }}</ref>. For example, if there are two groups of patients experiencing different treatment effects studies in two RCTs reporting conflicting results, the meta-analytic average is representative of neither group, similarly to averaging the weight of apples and oranges, which is neither accurate for apples nor oranges<ref>{{cite journal | vauthors = Feinstein AR | title = Meta-analysis: statistical alchemy for the 21st century | journal = Journal of Clinical Epidemiology | volume = 48 | issue = 1 | pages = 71–79 | date = January 1995 | pmid = 7853050 | doi = 10.1016/0895-4356(94)00110-C | series = The Potsdam International Consultation on Meta-Analysis }}</ref>. In performing a meta-analysis, an investigator must make choices which can affect the results, including deciding how to search for studies, selecting studies based on a set of objective criteria, dealing with incomplete data, analyzing the data, and accounting for or choosing not to account for [[publication bias]].<ref>{{cite journal | vauthors = Walker E, Hernandez AV, Kattan MW | title = Meta-analysis: Its strengths and limitations | journal = Cleveland Clinic Journal of Medicine | volume = 75 | issue = 6 | pages = 431–439 | date = June 2008 | pmid = 18595551 | doi = 10.3949/ccjm.75.6.431 }}</ref> This makes meta-analysis malleable in the sense that these methodological choices made in completing a meta-analysis are not determined but may affect the results<ref name="Stegenga" />. For instance, a meta-analysis of the same RCTs on the efficacy of local anesthesia and meta-regression of observational studies on the effects of meat consumption with, respectively, seven and eight meta-analytic techniques reported different p values of obtained average ATEs<ref>{{cite journal | vauthors = Guolo A, Varin C | title = Random-effects meta-analysis: the number of studies matters | journal = Statistical Methods in Medical Research | volume = 26 | issue = 3 | pages = 1500–1518 | date = June 2017 | pmid = 25953957 | doi = 10.1177/0962280215583568 | s2cid = 24423336 }}</ref>. For example, Wanous and colleagues examined four pairs of meta-analyses on the four topics of (a) job performance and satisfaction relationship, (b) realistic job previews, (c) correlates of role conflict and ambiguity, and (d) the job satisfaction and absenteeism relationship, and illustrated how various judgement calls made by the researchers produced different results.<ref>{{Cite journal| vauthors = Wanous JP, Sullivan SE, Malinak J |date=1989|title=The role of judgment calls in meta-analysis.|journal=Journal of Applied Psychology|volume=74|issue=2|pages=259–264|doi=10.1037/0021-9010.74.2.259|issn=0021-9010}}</ref> |
||
Meta-analyses are often, but not always, important components of a [[systematic review]] procedure. For instance, a meta-analysis may be conducted on several clinical trials of a medical treatment, in an effort to obtain a better understanding of how well the treatment works. Here it is convenient to follow the terminology used by the [[Cochrane Collaboration]],<ref>{{cite web|url=http://www.cochrane.org/glossary/|title=Glossary at Cochrane Collaboration|website=cochrane.org}}</ref> and use "meta-analysis" to refer to statistical methods of combining evidence, leaving other aspects of '[[research synthesis]]' or 'evidence synthesis', such as combining information from qualitative studies, for the more general context of systematic reviews. A meta-analysis is a [[secondary source]].<ref>{{Cite book|url=https://books.google.com/books?id=c69EDwAAQBAJ&pg=PA36|title=Research Methods for the Behavioral Sciences| |
Meta-analyses are often, but not always, important components of a [[systematic review]] procedure. For instance, a meta-analysis may be conducted on several clinical trials of a medical treatment, in an effort to obtain a better understanding of how well the treatment works. Here it is convenient to follow the terminology used by the [[Cochrane Collaboration]],<ref>{{cite web|url=http://www.cochrane.org/glossary/|title=Glossary at Cochrane Collaboration|website=cochrane.org}}</ref> and use "meta-analysis" to refer to statistical methods of combining evidence, leaving other aspects of '[[research synthesis]]' or 'evidence synthesis', such as combining information from qualitative studies, for the more general context of systematic reviews. A meta-analysis is a [[secondary source]].<ref>{{Cite book|url=https://books.google.com/books?id=c69EDwAAQBAJ&pg=PA36|title=Research Methods for the Behavioral Sciences| vauthors = Gravetter FJ, Forzano LB |date=2018-01-01|publisher=Cengage Learning|isbn=9781337613316|page=36|language=en|quote=Some examples of secondary sources are (1) books and textbooks in which the author describes and summarizes past research, (2) review articles or meta-analyses...}}</ref><ref>{{Cite book|url=https://books.google.com/books?id=K35ZDwAAQBAJ&pg=PT84|title=Research Methods, Statistics, and Applications| vauthors = Adams KA, Lawrence EK |date=2018-02-02|publisher=SAGE Publications|isbn=9781506350462|language=en|quote=The most common types of secondary sources found in academic journals are literature reviews and meta-analyses.}}</ref> In addition, meta-analysis may also be applied to a single study in cases where there are many cohorts which have not gone through identical selection criteria or to which the same investigational methologies have not been applied to all in the same manner or under the same exacting conditions. Under these circumstances each cohort is treated as an individual study and meta-analysis is used to draw study-wide conclusions.<ref>{{cite journal | vauthors = Rühlemann MC, Hermes BM, Bang C, Doms S, Moitinho-Silva L, Thingholm LB, Frost F, Degenhardt F, Wittig M, Kässens J, Weiss FU, Peters A, Neuhaus K, Völker U, Völzke H, Homuth G, Weiss S, Grallert H, Laudes M, Lieb W, Haller D, Lerch MM, Baines JF, Franke A | display-authors = 6 | title = Genome-wide association study in 8,956 German individuals identifies influence of ABO histo-blood groups on gut microbiome | journal = Nature Genetics | volume = 53 | issue = 2 | pages = 147–155 | date = February 2021 | pmid = 33462482 | doi = 10.1038/s41588-020-00747-1 | s2cid = 231641761 }}</ref> |
||
==History== |
==History== |
||
The historical roots of meta-analysis can be traced back to 17th century studies of astronomy,<ref name="Plackett1958">{{cite journal| |
The historical roots of meta-analysis can be traced back to 17th century studies of astronomy,<ref name="Plackett1958">{{cite journal| vauthors = Plackett RL |title=Studies in the History of Probability and Statistics: Vii. The Principle of the Arithmetic Mean|journal=Biometrika|date=1958|volume=45|issue=1–2|page=133|doi=10.1093/biomet/45.1-2.130}}</ref> while a paper published in 1904 by the statistician [[Karl Pearson]] in the ''[[British Medical Journal]]''<ref>{{cite journal | vauthors = Pearson K | title = Report on Certain Enteric Fever Inoculation Statistics | journal = British Medical Journal | volume = 2 | issue = 2288 | pages = 1243–1246 | date = November 1904 | pmid = 20761760 | pmc = 2355479 | doi = 10.1136/bmj.2.2288.1243 }}</ref> which collated data from several studies of typhoid inoculation is seen as the first time a meta-analytic approach was used to aggregate the outcomes of multiple clinical studies.<ref name=Nordmann2012>{{cite journal | vauthors = Nordmann AJ, Kasenda B, Briel M | title = Meta-analyses: what they can and cannot do | journal = Swiss Medical Weekly | volume = 142 | pages = w13518 | date = 9 March 2012 | pmid = 22407741 | doi = 10.4414/smw.2012.13518 | doi-access = free }}</ref><ref>{{cite journal | vauthors = O'Rourke K | title = An historical perspective on meta-analysis: dealing quantitatively with varying study results | journal = Journal of the Royal Society of Medicine | volume = 100 | issue = 12 | pages = 579–582 | date = December 2007 | pmid = 18065712 | pmc = 2121629 | doi = 10.1258/jrsm.100.12.579 }}</ref> The first meta-analysis of all conceptually identical experiments concerning a particular research issue, and conducted by independent researchers, has been identified as the 1940 book-length publication ''[[Extrasensory Perception After Sixty Years]]'', authored by Duke University psychologists [[Joseph Gaither Pratt|J. G. Pratt]], [[Joseph Banks Rhine|J. B. Rhine]], and associates.<ref>Pratt JG, Rhine JB, Smith BM, Stuart CE, Greenwood JA. Extra-Sensory Perception after Sixty Years: A Critical Appraisal of the Research in Extra-Sensory Perception. New York: Henry Holt, 1940</ref> This encompassed a review of 145 reports on [[Extrasensory perception|ESP]] experiments published from 1882 to 1939, and included an estimate of the influence of unpublished papers on the overall effect (the ''[[#Publication bias: the file drawer problem|file-drawer problem]]''). The term "meta-analysis" was coined in 1976 by the statistician [[Gene V. Glass]],<ref name="Glass">{{cite journal | vauthors = Glass GV |year=1976|title=Primary, secondary, and meta-analysis of research|journal=Educational Researcher|volume=5|issue=10|pages=3–8|doi=10.3102/0013189X005010003|s2cid=3185455}}</ref> who stated ''"my major interest currently is in what we have come to call ...the meta-analysis of research. The term is a bit grand, but it is precise and apt ... Meta-analysis refers to the analysis of analyses"''. Although this led to him being widely recognized as the modern founder of the method, the methodology behind what he termed "meta-analysis" predates his work by several decades.<ref>{{cite journal| vauthors = Cochran WG |year=1937 |title=Problems Arising in the Analysis of a Series of Similar Experiments|journal=Journal of the Royal Statistical Society|volume=4|issue=1|pages=102–118|doi=10.2307/2984123|jstor=2984123}}</ref><ref>{{cite journal|vauthors=Cochran WG, Carroll SP|year=1953|title=A Sampling Investigation of the Efficiency of Weighting Inversely as the Estimated Variance|journal=Biometrics|volume=9|issue=4|pages=447–459|doi=10.2307/3001436|jstor=3001436}}</ref> The statistical theory surrounding meta-analysis was greatly advanced by the work of [[Nambury S. Raju]], [[Larry V. Hedges]], Harris Cooper, [[Ingram Olkin]], [[John E. Hunter]], [[Jacob Cohen (born 1923)|Jacob Cohen]], [[Thomas C. Chalmers]], [[Robert Rosenthal (psychologist)|Robert Rosenthal]], [[Frank L. Schmidt]], [[John E. Hunter]], and Douglas G. Claurett.{{Clarify|reason=Not clear what each of these people has contributed to the field|date=July 2021}} In 1992, meta-analysis was first applied to ecological questions<ref>{{cite journal | vauthors = Gurevitch J, Koricheva J, Nakagawa S, Stewart G | title = Meta-analysis and the science of research synthesis | journal = Nature | volume = 555 | issue = 7695 | pages = 175–182 | date = March 2018 | pmid = 29517004 | doi = 10.1038/nature25753 | s2cid = 3761687 | bibcode = 2018Natur.555..175G }}</ref> by [[Jessica Gurevitch]] who used meta-analysis to study competition in field experiments.<ref>{{Cite journal| vauthors = Gurevitch J, Morrow LL, Wallace A, Walsh JS |date=1992|title=A Meta-Analysis of Competition in Field Experiments |journal=The American Naturalist|volume=140|issue=4|pages=539–572 |doi=10.1086/285428 |jstor=2462913 |s2cid=205982939 |issn=0003-0147}}</ref><ref name=":1">{{Cite web| vauthors = Gurevitch K |date=2018-03-07|title=Forty years of meta-analysis: We need evidence-based answers more than ever |url= https://researchdata.springernature.com/posts/30896-forty-years-of-meta-analysis-we-need-evidence-based-answers-more-than-ever |access-date=2021-07-24|website=Research Data at Springer Nature|language=en}}</ref> |
||
==Steps in a meta-analysis== |
==Steps in a meta-analysis== |
||
Line 51: | Line 51: | ||
AD is more commonly available (e.g. from the literature) and typically represents summary estimates such as odds ratios or relative risks. This can be directly synthesized across conceptually similar studies using several approaches (see below). On the other hand, indirect aggregate data measures the effect of two treatments that were each compared against a similar control group in a meta-analysis. For example, if treatment A and treatment B were directly compared vs placebo in separate meta-analyses, we can use these two pooled results to get an estimate of the effects of A vs B in an indirect comparison as effect A vs Placebo minus effect B vs Placebo. |
AD is more commonly available (e.g. from the literature) and typically represents summary estimates such as odds ratios or relative risks. This can be directly synthesized across conceptually similar studies using several approaches (see below). On the other hand, indirect aggregate data measures the effect of two treatments that were each compared against a similar control group in a meta-analysis. For example, if treatment A and treatment B were directly compared vs placebo in separate meta-analyses, we can use these two pooled results to get an estimate of the effects of A vs B in an indirect comparison as effect A vs Placebo minus effect B vs Placebo. |
||
IPD evidence represents raw data as collected by the study centers. This distinction has raised the need for different meta-analytic methods when evidence synthesis is desired, and has led to the development of one-stage and two-stage methods.<ref>{{ |
IPD evidence represents raw data as collected by the study centers. This distinction has raised the need for different meta-analytic methods when evidence synthesis is desired, and has led to the development of one-stage and two-stage methods.<ref>{{cite journal | vauthors = Debray TP, Moons KG, van Valkenhoef G, Efthimiou O, Hummel N, Groenwold RH, Reitsma JB | title = Get real in individual participant data (IPD) meta-analysis: a review of the methodology | journal = Research Synthesis Methods | volume = 6 | issue = 4 | pages = 293–309 | date = December 2015 | pmid = 26287812 | pmc = 5042043 | doi = 10.1002/jrsm.1160 }}</ref> In one-stage methods the IPD from all studies are modeled simultaneously whilst accounting for the clustering of participants within studies. Two-stage methods first compute summary statistics for AD from each study and then calculate overall statistics as a weighted average of the study statistics. By reducing IPD to AD, two-stage methods can also be applied when IPD is available; this makes them an appealing choice when performing a meta-analysis. Although it is conventionally believed that one-stage and two-stage methods yield similar results, recent studies have shown that they may occasionally lead to different conclusions.<ref name="pmid23585842">{{cite journal | vauthors = Debray TP, Moons KG, Abo-Zaid GM, Koffijberg H, Riley RD | title = Individual participant data meta-analysis for a binary outcome: one-stage or two-stage? | journal = PloS One | volume = 8 | issue = 4 | pages = e60650 | year = 2013 | pmid = 23585842 | pmc = 3621872 | doi = 10.1371/journal.pone.0060650 | doi-access = free | bibcode = 2013PLoSO...860650D }}</ref><ref>{{cite journal | vauthors = Burke DL, Ensor J, Riley RD | title = Meta-analysis using individual participant data: one-stage and two-stage approaches, and why they may differ | journal = Statistics in Medicine | volume = 36 | issue = 5 | pages = 855–875 | date = February 2017 | pmid = 27747915 | pmc = 5297998 | doi = 10.1002/sim.7141 }}</ref> |
||
===Statistical models for aggregate data=== |
===Statistical models for aggregate data=== |
||
Line 59: | Line 59: | ||
=====Fixed effects model===== |
=====Fixed effects model===== |
||
The fixed effect model provides a weighted average of a series of study estimates. The inverse of the estimates' variance is commonly used as study weight, so that larger studies tend to contribute more than smaller studies to the weighted average. Consequently, when studies within a meta-analysis are dominated by a very large study, the findings from smaller studies are practically ignored.<ref name="pmid11884693">{{cite journal | |
The fixed effect model provides a weighted average of a series of study estimates. The inverse of the estimates' variance is commonly used as study weight, so that larger studies tend to contribute more than smaller studies to the weighted average. Consequently, when studies within a meta-analysis are dominated by a very large study, the findings from smaller studies are practically ignored.<ref name="pmid11884693">{{cite journal | vauthors = Helfenstein U | title = Data and models determine treatment proposals--an illustration from meta-analysis | journal = Postgraduate Medical Journal | volume = 78 | issue = 917 | pages = 131–134 | date = March 2002 | pmid = 11884693 | pmc = 1742301 | doi = 10.1136/pmj.78.917.131 }}</ref> Most importantly, the fixed effects model assumes that all included studies investigate the same population, use the same variable and outcome definitions, etc. This assumption is typically unrealistic as research is often prone to several sources of [[study heterogeneity|heterogeneity]]; e.g. treatment effects may differ according to locale, dosage levels, study conditions, ... |
||
=====Random effects model===== |
=====Random effects model===== |
||
A common model used to synthesize [[study heterogeneity|heterogeneous]] research is the random effects model of meta-analysis. This is simply the weighted average of the effect sizes of a group of studies. The weight that is applied in this process of weighted averaging with a random effects meta-analysis is achieved in two steps:<ref>{{cite journal | |
A common model used to synthesize [[study heterogeneity|heterogeneous]] research is the random effects model of meta-analysis. This is simply the weighted average of the effect sizes of a group of studies. The weight that is applied in this process of weighted averaging with a random effects meta-analysis is achieved in two steps:<ref>{{cite journal | vauthors = Senn S | title = Trying to be precise about vagueness | journal = Statistics in Medicine | volume = 26 | issue = 7 | pages = 1417–1430 | date = March 2007 | pmid = 16906552 | doi = 10.1002/sim.2639 }}</ref> |
||
# Step 1: Inverse variance weighting |
# Step 1: Inverse variance weighting |
||
Line 70: | Line 70: | ||
This means that the greater this variability in effect sizes (otherwise known as [[study heterogeneity|heterogeneity]]), the greater the un-weighting and this can reach a point when the random effects meta-analysis result becomes simply the un-weighted average effect size across the studies. At the other extreme, when all effect sizes are similar (or variability does not exceed sampling error), no REVC is applied and the random effects meta-analysis defaults to simply a fixed effect meta-analysis (only inverse variance weighting). |
This means that the greater this variability in effect sizes (otherwise known as [[study heterogeneity|heterogeneity]]), the greater the un-weighting and this can reach a point when the random effects meta-analysis result becomes simply the un-weighted average effect size across the studies. At the other extreme, when all effect sizes are similar (or variability does not exceed sampling error), no REVC is applied and the random effects meta-analysis defaults to simply a fixed effect meta-analysis (only inverse variance weighting). |
||
The extent of this reversal is solely dependent on two factors:<ref name="ReferenceA">{{cite journal | vauthors = Al Khalaf MM, Thalib L, Doi SA |
The extent of this reversal is solely dependent on two factors:<ref name="ReferenceA">{{cite journal | vauthors = Al Khalaf MM, Thalib L, Doi SA | title = Combining heterogenous studies using the random-effects model is a mistake and leads to inconclusive meta-analyses | journal = Journal of Clinical Epidemiology | volume = 64 | issue = 2 | pages = 119–123 | date = February 2011 | pmid = 20409685 | doi = 10.1016/j.jclinepi.2010.01.009 }}</ref> |
||
# Heterogeneity of precision |
# Heterogeneity of precision |
||
Line 77: | Line 77: | ||
Since neither of these factors automatically indicates a faulty larger study or more reliable smaller studies, the re-distribution of weights under this model will not bear a relationship to what these studies actually might offer. Indeed, it has been demonstrated that redistribution of weights is simply in one direction from larger to smaller studies as heterogeneity increases until eventually all studies have equal weight and no more redistribution is possible.<ref name="ReferenceA"/> |
Since neither of these factors automatically indicates a faulty larger study or more reliable smaller studies, the re-distribution of weights under this model will not bear a relationship to what these studies actually might offer. Indeed, it has been demonstrated that redistribution of weights is simply in one direction from larger to smaller studies as heterogeneity increases until eventually all studies have equal weight and no more redistribution is possible.<ref name="ReferenceA"/> |
||
Another issue with the random effects model is that the most commonly used confidence intervals generally do not retain their coverage probability above the specified nominal level and thus substantially underestimate the statistical error and are potentially |
Another issue with the random effects model is that the most commonly used confidence intervals generally do not retain their coverage probability above the specified nominal level and thus substantially underestimate the statistical error and are potentially |
||
overconfident in their conclusions.<ref name="Brockwell2001">{{cite journal | |
overconfident in their conclusions.<ref name="Brockwell2001">{{cite journal | vauthors = Brockwell SE, Gordon IR | title = A comparison of statistical methods for meta-analysis | journal = Statistics in Medicine | volume = 20 | issue = 6 | pages = 825–840 | date = March 2001 | pmid = 11252006 | doi = 10.1002/sim.650 }}</ref><ref name="Noma2011">{{cite journal | vauthors = Noma H | title = Confidence intervals for a random-effects meta-analysis based on Bartlett-type corrections | journal = Statistics in Medicine | volume = 30 | issue = 28 | pages = 3304–3312 | date = December 2011 | pmid = 21964669 | doi = 10.1002/sim.4350 | hdl-access = free | hdl = 2433/152046 }}</ref> Several fixes have been suggested<ref>{{cite journal | vauthors = Brockwell SE, Gordon IR | title = A simple method for inference on an overall effect in meta-analysis | journal = Statistics in Medicine | volume = 26 | issue = 25 | pages = 4531–4543 | date = November 2007 | pmid = 17397112 | doi = 10.1002/sim.2883 }}</ref><ref>{{cite journal | vauthors = Sidik K, Jonkman JN | title = A simple confidence interval for meta-analysis | journal = Statistics in Medicine | volume = 21 | issue = 21 | pages = 3153–3159 | date = November 2002 | pmid = 12375296 | doi = 10.1002/sim.1262 }}</ref> but the debate continues on.<ref name="Noma2011" /><ref name="pmid19016302">{{cite journal | vauthors = Jackson D, Bowden J | title = A re-evaluation of the 'quantile approximation method' for random effects meta-analysis | journal = Statistics in Medicine | volume = 28 | issue = 2 | pages = 338–348 | date = January 2009 | pmid = 19016302 | pmc = 2991773 | doi = 10.1002/sim.3487 }}</ref> A further concern is that the average treatment effect can sometimes be even less conservative compared to the fixed effect model<ref>{{cite journal | vauthors = Poole C, Greenland S | title = Random-effects meta-analyses are not always conservative | journal = American Journal of Epidemiology | volume = 150 | issue = 5 | pages = 469–475 | date = September 1999 | pmid = 10472946 | doi = 10.1093/oxfordjournals.aje.a010035 | doi-access = free }}</ref> and therefore misleading in practice. One interpretational fix that has been suggested is to create a prediction interval around the random effects estimate to portray the range of possible effects in practice.<ref>{{cite journal | vauthors = Riley RD, Higgins JP, Deeks JJ | title = Interpretation of random effects meta-analyses | journal = BMJ | volume = 342 | pages = d549 | date = February 2011 | pmid = 21310794 | doi = 10.1136/bmj.d549 | s2cid = 32994689 }}</ref> However, an assumption behind the calculation of such a prediction interval is that trials are considered more or less homogeneous entities and that included patient populations and comparator treatments should be considered exchangeable<ref name="pmid23494781">{{cite journal | vauthors = Kriston L | title = Dealing with clinical heterogeneity in meta-analysis. Assumptions, methods, interpretation | journal = International Journal of Methods in Psychiatric Research | volume = 22 | issue = 1 | pages = 1–15 | date = March 2013 | pmid = 23494781 | pmc = 6878481 | doi = 10.1002/mpr.1377 }}</ref> and this is usually unattainable in practice. |
||
The most widely used method to estimate between studies variance (REVC) is the DerSimonian-Laird (DL) approach.<ref name="pmid3802833">{{cite journal |vauthors= |
The most widely used method to estimate between studies variance (REVC) is the DerSimonian-Laird (DL) approach.<ref name="pmid3802833">{{cite journal | vauthors = DerSimonian R, Laird N | title = Meta-analysis in clinical trials | journal = Controlled Clinical Trials | volume = 7 | issue = 3 | pages = 177–188 | date = September 1986 | pmid = 3802833 | doi = 10.1016/0197-2456(86)90046-2 }}</ref> Several advanced iterative (and computationally expensive) techniques for computing the between studies variance exist (such as maximum likelihood, profile likelihood and restricted maximum likelihood methods) and random effects models using these methods can be run in Stata with the metaan command.<ref name="metaan">{{cite journal|url=https://www.researchgate.net/publication/227629391|title=Metaan: Random-effects meta-analysis| vauthors = Kontopantelis E, Reeves D |date=1 August 2010|journal=Stata Journal|volume=10|issue=3|pages=395–407|via=ResearchGate |doi= 10.1177/1536867X1001000307 |doi-access=free}}</ref> The metaan command must be distinguished from the classic metan (single "a") command in Stata that uses the DL estimator. These advanced methods have also been implemented in a free and easy to use Microsoft Excel add-on, MetaEasy.<ref name="MetaEasy1">{{cite journal|title=MetaEasy:A Meta-Analysis Add-In for Microsoft Excel, Journal of Statistical Software 2009 |journal=Journal of Statistical Software |volume=30 |issue=7 |doi=10.18637/jss.v030.i07 |year=2009 | vauthors = Kontopantelis E, Reeves D |doi-access=free }}</ref><ref name="MetaEasy2">{{cite web|url=http://www.statanalysis.co.uk/meta-analysis.html |title=Developer's website |publisher=Statanalysis.co.uk |access-date=2018-09-18}}</ref> However, a comparison between these advanced methods and the DL method of computing the between studies variance demonstrated that there is little to gain and DL is quite adequate in most scenarios.<ref name=KontopantelisSMMR10>{{cite journal | vauthors = Kontopantelis E, Reeves D | title = Performance of statistical methods for meta-analysis when true study effects are non-normally distributed: A simulation study | journal = Statistical Methods in Medical Research | volume = 21 | issue = 4 | pages = 409–426 | date = August 2012 | pmid = 21148194 | doi = 10.1177/0962280210392008 | s2cid = 152379 }}</ref><ref name=KontopantelisSMMR11>{{cite journal | vauthors = Kontopantelis E, Reeves D | title = Performance of statistical methods for meta-analysis when true study effects are non-normally distributed: a comparison between DerSimonian-Laird and restricted maximum likelihood | journal = Statistical Methods in Medical Research | volume = 21 | issue = 6 | pages = 657–659 | date = December 2012 | pmid = 23171971 | doi = 10.1177/0962280211413451 | s2cid = 44928009 }}</ref> |
||
⚫ | However, most meta-analyses include between 2 and 4 studies and such a sample is more often than not inadequate to accurately estimate [[study heterogeneity|heterogeneity]]. Thus it appears that in small meta-analyses, an incorrect zero between study variance estimate is obtained, leading to a false homogeneity assumption. Overall, it appears that heterogeneity is being consistently underestimated in meta-analyses and sensitivity analyses in which high heterogeneity levels are assumed could be informative.<ref name=KontopantelisP1>{{cite journal |vauthors=Kontopantelis E, Springate DA, Reeves D | title = A |
||
⚫ | However, most meta-analyses include between 2 and 4 studies and such a sample is more often than not inadequate to accurately estimate [[study heterogeneity|heterogeneity]]. Thus it appears that in small meta-analyses, an incorrect zero between study variance estimate is obtained, leading to a false homogeneity assumption. Overall, it appears that heterogeneity is being consistently underestimated in meta-analyses and sensitivity analyses in which high heterogeneity levels are assumed could be informative.<ref name=KontopantelisP1>{{cite journal | vauthors = Kontopantelis E, Springate DA, Reeves D | title = A re-analysis of the Cochrane Library data: the dangers of unobserved heterogeneity in meta-analyses | journal = PloS One | volume = 8 | issue = 7 | pages = e69930 | year = 2013 | pmid = 23922860 | pmc = 3724681 | doi = 10.1371/journal.pone.0069930 | veditors = Friede T | doi-access = free | bibcode = 2013PLoSO...869930K }}</ref> These random effects models and software packages mentioned above relate to study-aggregate meta-analyses and researchers wishing to conduct individual patient data (IPD) meta-analyses need to consider mixed-effects modelling approaches.<ref name="ipdforest">{{cite journal |url= https://www.researchgate.net/publication/257316967 |title=A short guide and a forest plot command (ipdforest) for one-stage meta-analysis| vauthors = Kontopantelis E, Reeves D |date=27 September 2013|journal=Stata Journal|volume=13|issue=3|pages=574–587 |via= ResearchGate |doi=10.1177/1536867X1301300308 |doi-access=free}}</ref> |
||
=====IVhet model===== |
=====IVhet model===== |
||
Doi & Barendregt working in collaboration with Khan, Thalib and Williams (from the University of Queensland, University of Southern Queensland and Kuwait University), have created an inverse variance quasi likelihood based alternative (IVhet) to the random effects (RE) model for which details are available online.<ref name="Manual">{{cite web|url=http://www.epigear.com/index_files/MetaXL%20User%20Guide.pdf |title=MetaXL User Guide |access-date=2018-09-18}}</ref> This was incorporated into MetaXL version 2.0,<ref name="Epigear" /> a free Microsoft excel add-in for meta-analysis produced by Epigear International Pty Ltd, and made available on 5 April 2014. The authors state that a clear advantage of this model is that it resolves the two main problems of the random effects model. The first advantage of the IVhet model is that coverage remains at the nominal (usually 95%) level for the confidence interval unlike the random effects model which drops in coverage with increasing heterogeneity.<ref name="Brockwell2001" /><ref name="Noma2011" /> The second advantage is that the IVhet model maintains the inverse variance weights of individual studies, unlike the RE model which gives small studies more weight (and therefore larger studies less) with increasing heterogeneity. When heterogeneity becomes large, the individual study weights under the RE model become equal and thus the RE model returns an arithmetic mean rather than a weighted average. This side-effect of the RE model does not occur with the IVhet model which thus differs from the RE model estimate in two perspectives:<ref name="Manual" /> Pooled estimates will favor larger trials (as opposed to penalizing larger trials in the RE model) and will have a confidence interval that remains within the nominal coverage under uncertainty (heterogeneity). Doi & Barendregt suggest that while the RE model provides an alternative method of pooling the study data, their simulation results<ref>{{cite journal |vauthors=Doi SA, Barendregt JJ, Khan S, Thalib L, Williams GM |
Doi & Barendregt working in collaboration with Khan, Thalib and Williams (from the University of Queensland, University of Southern Queensland and Kuwait University), have created an inverse variance quasi likelihood based alternative (IVhet) to the random effects (RE) model for which details are available online.<ref name="Manual">{{cite web|url=http://www.epigear.com/index_files/MetaXL%20User%20Guide.pdf |title=MetaXL User Guide |access-date=2018-09-18}}</ref> This was incorporated into MetaXL version 2.0,<ref name="Epigear" /> a free Microsoft excel add-in for meta-analysis produced by Epigear International Pty Ltd, and made available on 5 April 2014. The authors state that a clear advantage of this model is that it resolves the two main problems of the random effects model. The first advantage of the IVhet model is that coverage remains at the nominal (usually 95%) level for the confidence interval unlike the random effects model which drops in coverage with increasing heterogeneity.<ref name="Brockwell2001" /><ref name="Noma2011" /> The second advantage is that the IVhet model maintains the inverse variance weights of individual studies, unlike the RE model which gives small studies more weight (and therefore larger studies less) with increasing heterogeneity. When heterogeneity becomes large, the individual study weights under the RE model become equal and thus the RE model returns an arithmetic mean rather than a weighted average. This side-effect of the RE model does not occur with the IVhet model which thus differs from the RE model estimate in two perspectives:<ref name="Manual" /> Pooled estimates will favor larger trials (as opposed to penalizing larger trials in the RE model) and will have a confidence interval that remains within the nominal coverage under uncertainty (heterogeneity). Doi & Barendregt suggest that while the RE model provides an alternative method of pooling the study data, their simulation results<ref>{{cite journal | vauthors = Doi SA, Barendregt JJ, Khan S, Thalib L, Williams GM | title = Advances in the meta-analysis of heterogeneous clinical trials I: The inverse variance heterogeneity model | journal = Contemporary Clinical Trials | volume = 45 | issue = Pt A | pages = 130–138 | date = November 2015 | pmid = 26003435 | doi = 10.1016/j.cct.2015.05.009 | hdl-access = free | hdl = 1885/17083 }}</ref> demonstrate that using a more specified probability model with untenable assumptions, as with the RE model, does not necessarily provide better results. The latter study also reports that the IVhet model resolves the problems related to underestimation of the statistical error, poor coverage of the confidence interval and increased MSE seen with the random effects model and the authors conclude that researchers should henceforth abandon use of the random effects model in meta-analysis. While their data is compelling, the ramifications (in terms of the magnitude of spuriously positive results within the Cochrane database) are huge and thus accepting this conclusion requires careful independent confirmation. The availability of a free software (MetaXL)<ref name="Epigear" /> that runs the IVhet model (and all other models for comparison) facilitates this for the research community. |
||
==== Direct evidence: Models incorporating additional information ==== |
==== Direct evidence: Models incorporating additional information ==== |
||
Line 91: | Line 91: | ||
=====Quality effects model===== |
=====Quality effects model===== |
||
Doi and Thalib originally introduced the quality effects model.<ref name="Doi_2008">{{cite journal |vauthors=Doi SA, Thalib L | title = A quality-effects model for meta-analysis | journal = Epidemiology | volume = 19 | issue = 1 | pages = 94–100 | |
Doi and Thalib originally introduced the quality effects model.<ref name="Doi_2008">{{cite journal | vauthors = Doi SA, Thalib L | title = A quality-effects model for meta-analysis | journal = Epidemiology | volume = 19 | issue = 1 | pages = 94–100 | date = January 2008 | pmid = 18090860 | doi = 10.1097/EDE.0b013e31815c24e7 | s2cid = 29723291 }}</ref> They<ref>{{cite journal | vauthors = Doi SA, Barendregt JJ, Mozurkewich EL | title = Meta-analysis of heterogeneous clinical trials: an empirical example | journal = Contemporary Clinical Trials | volume = 32 | issue = 2 | pages = 288–298 | date = March 2011 | pmid = 21147265 | doi = 10.1016/j.cct.2010.12.006 }}</ref> introduced a new approach to adjustment for inter-study variability by incorporating the contribution of variance due to a relevant component (quality) in addition to the contribution of variance due to random error that is used in any fixed effects meta-analysis model to generate weights for each study. The strength of the quality effects meta-analysis is that it allows available methodological evidence to be used over subjective random effects, and thereby helps to close the damaging gap which has opened up between methodology and statistics in clinical research. To do this a synthetic bias variance is computed based on quality information to adjust inverse variance weights and the quality adjusted weight of the ''i''th study is introduced.<ref name="Doi_2008"/> These adjusted weights are then used in meta-analysis. In other words, if study ''i'' is of good quality and other studies are of poor quality, a proportion of their quality adjusted weights is mathematically redistributed to study ''i'' giving it more weight towards the overall effect size. As studies become increasingly similar in terms of quality, re-distribution becomes progressively less and ceases when all studies are of equal quality (in the case of equal quality, the quality effects model defaults to the IVhet model – see previous section). A recent evaluation of the quality effects model (with some updates) demonstrates that despite the subjectivity of quality assessment, the performance (MSE and true variance under simulation) is superior to that achievable with the random effects model.<ref>{{cite journal | vauthors = Doi SA, Barendregt JJ, Khan S, Thalib L, Williams GM | title = Simulation Comparison of the Quality Effects and Random Effects Methods of Meta-analysis | journal = Epidemiology | volume = 26 | issue = 4 | pages = e42-e44 | date = July 2015 | pmid = 25872162 | doi = 10.1097/EDE.0000000000000289 }}</ref><ref>{{cite journal | vauthors = Doi SA, Barendregt JJ, Khan S, Thalib L, Williams GM | title = Advances in the meta-analysis of heterogeneous clinical trials II: The quality effects model | journal = Contemporary Clinical Trials | volume = 45 | issue = Pt A | pages = 123–129 | date = November 2015 | pmid = 26003432 | doi = 10.1016/j.cct.2015.05.010 }}</ref> This model thus replaces the untenable interpretations that abound in the literature and a software is available to explore this method further.<ref name="Epigear">{{cite web|url=http://www.epigear.com/ |title=MetaXL software page |publisher=Epigear.com |date=2017-06-03 |access-date=2018-09-18}}</ref> |
||
==== Indirect evidence: Network meta-analysis methods ==== |
==== Indirect evidence: Network meta-analysis methods ==== |
||
[[File:Indirekt jämförelse.jpg|thumb|300px|right|A network meta-analysis looks at indirect comparisons. In the image, A has been analyzed in relation to C and C has been analyzed in relation to b. However the relation between A and B is only known indirectly, and a network meta-analysis looks at such indirect evidence of differences between methods and interventions using statistical method.]] |
[[File:Indirekt jämförelse.jpg|thumb|300px|right|A network meta-analysis looks at indirect comparisons. In the image, A has been analyzed in relation to C and C has been analyzed in relation to b. However the relation between A and B is only known indirectly, and a network meta-analysis looks at such indirect evidence of differences between methods and interventions using statistical method.]] |
||
Indirect comparison meta-analysis methods (also called network meta-analyses, in particular when multiple treatments are assessed simultaneously) generally use two main methodologies. First, is the Bucher method<ref>{{cite journal | |
Indirect comparison meta-analysis methods (also called network meta-analyses, in particular when multiple treatments are assessed simultaneously) generally use two main methodologies. First, is the Bucher method<ref>{{cite journal | vauthors = Bucher HC, Guyatt GH, Griffith LE, Walter SD | title = The results of direct and indirect treatment comparisons in meta-analysis of randomized controlled trials | journal = Journal of Clinical Epidemiology | volume = 50 | issue = 6 | pages = 683–691 | date = June 1997 | pmid = 9250266 | doi = 10.1016/s0895-4356(97)00049-8 }}</ref> which is a single or repeated comparison of a closed loop of three-treatments such that one of them is common to the two studies and forms the node where the loop begins and ends. Therefore, multiple two-by-two comparisons (3-treatment loops) are needed to compare multiple treatments. This methodology requires that trials with more than two arms have two arms only selected as independent pair-wise comparisons are required. The alternative methodology uses complex [[statistical model]]ling to include the multiple arm trials and comparisons simultaneously between all competing treatments. These have been executed using Bayesian methods, mixed linear models and meta-regression approaches.{{citation needed|date=June 2018}} |
||
=====Bayesian framework===== |
=====Bayesian framework===== |
||
Specifying a Bayesian network meta-analysis model involves writing a directed acyclic graph (DAG) model for general-purpose [[Markov chain Monte Carlo]] (MCMC) software such as WinBUGS.<ref name="Valkenhoef, G. 2012">{{cite journal | |
Specifying a Bayesian network meta-analysis model involves writing a directed acyclic graph (DAG) model for general-purpose [[Markov chain Monte Carlo]] (MCMC) software such as WinBUGS.<ref name="Valkenhoef, G. 2012">{{cite journal | vauthors = van Valkenhoef G, Lu G, de Brock B, Hillege H, Ades AE, Welton NJ | title = Automating network meta-analysis | journal = Research Synthesis Methods | volume = 3 | issue = 4 | pages = 285–299 | date = December 2012 | pmid = 26053422 | doi = 10.1002/jrsm.1054 }}</ref> In addition, prior distributions have to be specified for a number of the parameters, and the data have to be supplied in a specific format.<ref name="Valkenhoef, G. 2012"/> Together, the DAG, priors, and data form a Bayesian hierarchical model. To complicate matters further, because of the nature of MCMC estimation, overdispersed starting values have to be chosen for a number of independent chains so that convergence can be assessed.<ref>{{cite journal |vauthors=Brooks SP, Gelman A | year = 1998 | title = General methods for monitoring convergence of iterative simulations | url = http://www.stat.columbia.edu/~gelman/research/published/brooksgelman2.pdf| journal = Journal of Computational and Graphical Statistics | volume = 7 | issue = 4| pages = 434–455 | doi=10.1080/10618600.1998.10474787}}</ref> Currently, there is no software that automatically generates such models, although there are some tools to aid in the process. The complexity of the Bayesian approach has limited usage of this methodology. Methodology for automation of this method has been suggested<ref>van Valkenhoef G, Lu G, de Brock B, Hillege H, Ades AE, Welton NJ. Automating network meta-analysis. Res Synth Methods. 2012 Dec;3(4):285-99.</ref> but requires that arm-level outcome data are available, and this is usually unavailable. Great claims are sometimes made for the inherent ability of the Bayesian framework to handle network meta-analysis and its greater flexibility. However, this choice of implementation of framework for inference, Bayesian or frequentist, may be less important than other choices regarding the modeling of effects<ref name="ReferenceC">{{cite journal | vauthors = Senn S, Gavini F, Magrez D, Scheen A | title = Issues in performing a network meta-analysis | journal = Statistical Methods in Medical Research | volume = 22 | issue = 2 | pages = 169–189 | date = April 2013 | pmid = 22218368 | doi = 10.1177/0962280211432220 | s2cid = 10860031 }}</ref> (see discussion on models above). |
||
=====Frequentist multivariate framework===== |
=====Frequentist multivariate framework===== |
||
On the other hand, the frequentist multivariate methods involve approximations and assumptions that are not stated explicitly or verified when the methods are applied (see discussion on meta-analysis models above). For example, the mvmeta package for Stata enables network meta-analysis in a frequentist framework.<ref>{{cite journal | |
On the other hand, the frequentist multivariate methods involve approximations and assumptions that are not stated explicitly or verified when the methods are applied (see discussion on meta-analysis models above). For example, the mvmeta package for Stata enables network meta-analysis in a frequentist framework.<ref>{{cite journal | vauthors = White IR | year = 2011 | title = Multivariate random-effects meta-regression: updates to mvmeta | journal = The Stata Journal | volume = 11 | issue = 2| pages = 255–270| doi = 10.1177/1536867X1101100206 | doi-access = free }}</ref> However, if there is no common comparator in the network, then this has to be handled by augmenting the dataset with fictional arms with high variance, which is not very objective and requires a decision as to what constitutes a sufficiently high variance.<ref>van Valkenhoef G, Lu G, de Brock B, Hillege H, Ades AE, Welton NJ. Automating network meta-analysis. Res Synth Methods. 2012 Dec;3(4):285-99</ref> The other issue is use of the random effects model in both this frequentist framework and the Bayesian framework. Senn advises analysts to be cautious about interpreting the 'random effects' analysis since only one random effect is allowed for but one could envisage many.<ref name="ReferenceC"/> Senn goes on to say that it is rather naıve, even in the case where only two treatments are being compared to assume that random-effects analysis accounts for all uncertainty about the way effects can vary from trial to trial. Newer models of meta-analysis such as those discussed above would certainly help alleviate this situation and have been implemented in the next framework. |
||
=====Generalized pairwise modelling framework===== |
=====Generalized pairwise modelling framework===== |
||
Line 108: | Line 108: | ||
=====Tailored meta-analysis===== |
=====Tailored meta-analysis===== |
||
Another form of additional information comes from the intended setting. If the target setting for applying the meta-analysis results is known then it may be possible to use data from the setting to tailor the results thus producing a 'tailored meta-analysis'.,<ref>{{cite journal |vauthors=Willis BH, Hyde CJ |
Another form of additional information comes from the intended setting. If the target setting for applying the meta-analysis results is known then it may be possible to use data from the setting to tailor the results thus producing a 'tailored meta-analysis'.,<ref>{{cite journal | vauthors = Willis BH, Hyde CJ | title = Estimating a test's accuracy using tailored meta-analysis-How setting-specific data may aid study selection | journal = Journal of Clinical Epidemiology | volume = 67 | issue = 5 | pages = 538–546 | date = May 2014 | pmid = 24447592 | doi = 10.1016/j.jclinepi.2013.10.016 }}</ref><ref>{{cite journal | vauthors = Willis BH, Hyde CJ | title = What is the test's accuracy in my practice population? Tailored meta-analysis provides a plausible estimate | journal = Journal of Clinical Epidemiology | volume = 68 | issue = 8 | pages = 847–854 | date = August 2015 | pmid = 25479685 | doi = 10.1016/j.jclinepi.2014.10.002 }}</ref> This has been used in test accuracy meta-analyses, where empirical knowledge of the test positive rate and the prevalence have been used to derive a region in [[Receiver Operating Characteristic]] (ROC) space known as an 'applicable region'. Studies are then selected for the target setting based on comparison with this region and aggregated to produce a summary estimate which is tailored to the target setting. |
||
==== Aggregating IPD and AD ==== |
==== Aggregating IPD and AD ==== |
||
Line 114: | Line 114: | ||
===Validation of meta-analysis results=== |
===Validation of meta-analysis results=== |
||
The meta-analysis estimate represents a weighted average across studies and when there is [[study heterogeneity|heterogeneity]] this may result in the summary estimate not being representative of individual studies. Qualitative appraisal of the primary studies using established tools can uncover potential biases,<ref>{{cite journal | |
The meta-analysis estimate represents a weighted average across studies and when there is [[study heterogeneity|heterogeneity]] this may result in the summary estimate not being representative of individual studies. Qualitative appraisal of the primary studies using established tools can uncover potential biases,<ref>{{cite journal | vauthors = Higgins JP, Altman DG, Gøtzsche PC, Jüni P, Moher D, Oxman AD, Savovic J, Schulz KF, Weeks L, Sterne JA | display-authors = 6 | title = The Cochrane Collaboration's tool for assessing risk of bias in randomised trials | journal = BMJ | volume = 343 | pages = d5928 | date = October 2011 | pmid = 22008217 | pmc = 3196245 | doi = 10.1136/bmj.d5928 }}</ref><ref>{{cite journal | vauthors = Whiting PF, Rutjes AW, Westwood ME, Mallett S, Deeks JJ, Reitsma JB, Leeflang MM, Sterne JA, Bossuyt PM | display-authors = 6 | title = QUADAS-2: a revised tool for the quality assessment of diagnostic accuracy studies | journal = Annals of Internal Medicine | volume = 155 | issue = 8 | pages = 529–536 | date = October 2011 | pmid = 22007046 | doi = 10.7326/0003-4819-155-8-201110180-00009 | doi-access = free }}</ref> but does not quantify the aggregate effect of these biases on the summary estimate. Although the meta-analysis result could be compared with an independent prospective primary study, such external validation is often impractical. This has led to the development of methods that exploit a form of [[Cross-validation (statistics)|leave-one-out cross validation]], sometimes referred to as internal-external cross validation (IOCV).<ref>{{cite journal | vauthors = Royston P, Parmar MK, Sylvester R | title = Construction and validation of a prognostic model across several studies, with an application in superficial bladder cancer | journal = Statistics in Medicine | volume = 23 | issue = 6 | pages = 907–926 | date = March 2004 | pmid = 15027080 | doi = 10.1002/sim.1691 }}</ref> Here each of the k included studies in turn is omitted and compared with the summary estimate derived from aggregating the remaining k- 1 studies. A general '''validation statistic, Vn''' based on IOCV has been developed to measure the statistical validity of meta-analysis results.<ref>{{cite journal | vauthors = Willis BH, Riley RD | title = Measuring the statistical validity of summary meta-analysis and meta-regression results for use in clinical practice | journal = Statistics in Medicine | volume = 36 | issue = 21 | pages = 3283–3301 | date = September 2017 | pmid = 28620945 | pmc = 5575530 | doi = 10.1002/sim.7372 }}</ref> For test accuracy and prediction, particularly when there are multivariate effects, other approaches which seek to estimate the prediction error have also been proposed.<ref>{{cite journal | vauthors = Riley RD, Ahmed I, Debray TP, Willis BH, Noordzij JP, Higgins JP, Deeks JJ | title = Summarising and validating test accuracy results across multiple studies for use in clinical practice | journal = Statistics in Medicine | volume = 34 | issue = 13 | pages = 2081–2103 | date = June 2015 | pmid = 25800943 | pmc = 4973708 | doi = 10.1002/sim.6471 }}</ref> |
||
==Challenges== |
==Challenges== |
||
A meta-analysis of several small studies does not always predict the results of a single large study.<ref>{{cite journal |vauthors=LeLorier J, Grégoire G, Benhaddad A, Lapierre J, Derderian F | title = Discrepancies between |
A meta-analysis of several small studies does not always predict the results of a single large study.<ref>{{cite journal | vauthors = LeLorier J, Grégoire G, Benhaddad A, Lapierre J, Derderian F | title = Discrepancies between meta-analyses and subsequent large randomized, controlled trials | journal = The New England Journal of Medicine | volume = 337 | issue = 8 | pages = 536–542 | date = August 1997 | pmid = 9262498 | doi = 10.1056/NEJM199708213370806 }}</ref> Some have argued that a weakness of the method is that sources of bias are not controlled by the method: a good meta-analysis cannot correct for poor design or bias in the original studies.<ref name=Slavin>{{cite journal | vauthors = Slavin RE | title = Best-Evidence Synthesis: An Alternative to Meta-Analytic and Traditional Reviews | journal = Educational Researcher | volume = 15 | issue = 9 | pages = 5–9 | year = 1986 | doi = 10.3102/0013189X015009005| s2cid = 146457142 }}</ref> This would mean that only methodologically sound studies should be included in a meta-analysis, a practice called 'best evidence synthesis'.<ref name=Slavin/> Other meta-analysts would include weaker studies, and add a study-level predictor variable that reflects the methodological quality of the studies to examine the effect of study quality on the effect size.<ref>{{cite book | vauthors = Hunter JE, Schmidt FL, Jackson GB | collaboration = American Psychological Association. Division of Industrial-Organizational Psychology |title=Meta-analysis: cumulating research findings across studies |date=1982 |publisher=Sage |location=Beverly Hills, California |isbn=978-0-8039-1864-1}}</ref> However, others have argued that a better approach is to preserve information about the variance in the study sample, casting as wide a net as possible, and that methodological selection criteria introduce unwanted subjectivity, defeating the purpose of the approach.<ref>{{cite book | vauthors = Glass GV, McGaw B, Smith ML|title=Meta-analysis in social research |date=1981 |publisher=Sage Publications |location=Beverly Hills, California |isbn=978-0-8039-1633-3}}</ref> |
||
===Publication bias: the file drawer problem=== |
===Publication bias: the file drawer problem=== |
||
[[File:Example of a symmetrical funnel plot created with MetaXL Sept 2015.jpg|thumb|right|A funnel plot expected without the file drawer problem. The largest studies converge at the tip while smaller studies show more or less symmetrical scatter at the base]] |
[[File:Example of a symmetrical funnel plot created with MetaXL Sept 2015.jpg|thumb|right|A funnel plot expected without the file drawer problem. The largest studies converge at the tip while smaller studies show more or less symmetrical scatter at the base]] |
||
[[File:Funnel plot depicting asymmetry Sept 2015.jpg|thumb|right|A funnel plot expected with the file drawer problem. The largest studies still cluster around the tip, but the bias against publishing negative studies has caused the smaller studies as a whole to have an unjustifiably favorable result to the hypothesis]] |
[[File:Funnel plot depicting asymmetry Sept 2015.jpg|thumb|right|A funnel plot expected with the file drawer problem. The largest studies still cluster around the tip, but the bias against publishing negative studies has caused the smaller studies as a whole to have an unjustifiably favorable result to the hypothesis]] |
||
Another potential pitfall is the reliance on the available body of published studies, which may create exaggerated outcomes due to [[publication bias]], as studies which show [[Null result|negative results]] or [[statistically insignificant|insignificant]] results are less likely to be published. For example, pharmaceutical companies have been known to hide negative studies and researchers may have overlooked unpublished studies such as dissertation studies or conference abstracts that did not reach publication. This is not easily solved, as one cannot know how many studies have gone unreported.<ref name=Rosenthal1979>{{cite journal |doi=10.1037/0033-2909.86.3.638 |year=1979 | |
Another potential pitfall is the reliance on the available body of published studies, which may create exaggerated outcomes due to [[publication bias]], as studies which show [[Null result|negative results]] or [[statistically insignificant|insignificant]] results are less likely to be published. For example, pharmaceutical companies have been known to hide negative studies and researchers may have overlooked unpublished studies such as dissertation studies or conference abstracts that did not reach publication. This is not easily solved, as one cannot know how many studies have gone unreported.<ref name=Rosenthal1979>{{cite journal |doi=10.1037/0033-2909.86.3.638 |year=1979 | vauthors = Rosenthal R |author-link=Robert Rosenthal (psychologist) |title=The "File Drawer Problem" and the Tolerance for Null Results |journal=[[Psychological Bulletin]] |volume=86 |issue=3 |pages=638–641|s2cid=36070395 |url=https://semanticscholar.org/paper/dfa3c3689503600fe0bcc45eb0ad319e313079b9 }}</ref> |
||
This [[file drawer problem]] (characterized by negative or non-significant results being tucked away in a cabinet), can result in a biased distribution of effect sizes thus creating a serious [[base rate fallacy]], in which the significance of the published studies is overestimated, as other studies were either not submitted for publication or were rejected. This should be seriously considered when interpreting the outcomes of a meta-analysis.<ref name=Rosenthal1979/><ref name=Hunter&Schmidt1990>{{Cite book|year=1990 | |
This [[file drawer problem]] (characterized by negative or non-significant results being tucked away in a cabinet), can result in a biased distribution of effect sizes thus creating a serious [[base rate fallacy]], in which the significance of the published studies is overestimated, as other studies were either not submitted for publication or were rejected. This should be seriously considered when interpreting the outcomes of a meta-analysis.<ref name=Rosenthal1979/><ref name=Hunter&Schmidt1990>{{Cite book|year=1990 | vauthors = Hunter JE, Schmidt FL |author-link1=John E. Hunter |author-link2=Frank L. Schmidt |title=Methods of Meta-Analysis: Correcting Error and Bias in Research Findings |place=Newbury Park, California; London; New Delhi |publisher=[[SAGE Publications]]}}</ref> |
||
The distribution of effect sizes can be visualized with a [[funnel plot]] which (in its most common version) is a scatter plot of standard error versus the effect size. It makes use of the fact that the smaller studies (thus larger standard errors) have more scatter of the magnitude of effect (being less precise) while the larger studies have less scatter and form the tip of the funnel. If many negative studies were not published, the remaining positive studies give rise to a funnel plot in which the base is skewed to one side (asymmetry of the funnel plot). In contrast, when there is no publication bias, the effect of the smaller studies has no reason to be skewed to one side and so a symmetric funnel plot results. This also means that if no publication bias is present, there would be no relationship between standard error and effect size.<ref>{{cite book| |
The distribution of effect sizes can be visualized with a [[funnel plot]] which (in its most common version) is a scatter plot of standard error versus the effect size. It makes use of the fact that the smaller studies (thus larger standard errors) have more scatter of the magnitude of effect (being less precise) while the larger studies have less scatter and form the tip of the funnel. If many negative studies were not published, the remaining positive studies give rise to a funnel plot in which the base is skewed to one side (asymmetry of the funnel plot). In contrast, when there is no publication bias, the effect of the smaller studies has no reason to be skewed to one side and so a symmetric funnel plot results. This also means that if no publication bias is present, there would be no relationship between standard error and effect size.<ref>{{cite book | vauthors = Light RJ, Pillemer DB |title=Summing up : the science of reviewing research |date=1984 |publisher=Harvard University Press |location=Cambridge, Massachusetts |isbn=978-0-674-85431-4 |url=https://archive.org/details/summingupscience00ligh }}</ref> A negative or positive relation between standard error and effect size would imply that smaller studies that found effects in one direction only were more likely to be published and/or to be submitted for publication. |
||
Apart from the visual funnel plot, statistical methods for detecting publication bias have also been proposed. These are controversial because they typically have low power for detection of bias, but also may make false positives under some circumstances.<ref name="Ioannidis and Trikalinos">{{cite journal |vauthors=Ioannidis JP, Trikalinos TA | title = The appropriateness of asymmetry tests for publication bias in meta-analyses: a large survey | journal = |
Apart from the visual funnel plot, statistical methods for detecting publication bias have also been proposed. These are controversial because they typically have low power for detection of bias, but also may make false positives under some circumstances.<ref name="Ioannidis and Trikalinos">{{cite journal | vauthors = Ioannidis JP, Trikalinos TA | title = The appropriateness of asymmetry tests for publication bias in meta-analyses: a large survey | journal = Cmaj | volume = 176 | issue = 8 | pages = 1091–1096 | date = April 2007 | pmid = 17420491 | pmc = 1839799 | doi = 10.1503/cmaj.060410 }}</ref> For instance small study effects (biased smaller studies), wherein methodological differences between smaller and larger studies exist, may cause asymmetry in effect sizes that resembles publication bias. However, small study effects may be just as problematic for the interpretation of meta-analyses, and the imperative is on meta-analytic authors to investigate potential sources of bias. |
||
A Tandem Method for analyzing publication bias has been suggested for cutting down false positive error problems.<ref name="Ferguson and Brannick">{{cite journal |vauthors=Ferguson CJ, Brannick MT | title = Publication bias in psychological science: prevalence, methods for identifying and controlling, and implications for the use of meta-analyses | journal = |
A Tandem Method for analyzing publication bias has been suggested for cutting down false positive error problems.<ref name="Ferguson and Brannick">{{cite journal | vauthors = Ferguson CJ, Brannick MT | title = Publication bias in psychological science: prevalence, methods for identifying and controlling, and implications for the use of meta-analyses | journal = Psychological Methods | volume = 17 | issue = 1 | pages = 120–128 | date = March 2012 | pmid = 21787082 | doi = 10.1037/a0024445 }}</ref> This Tandem method consists of three stages. Firstly, one calculates Orwin's fail-safe N, to check how many studies should be added in order to reduce the test statistic to a trivial size. If this number of studies is larger than the number of studies used in the meta-analysis, it is a sign that there is no publication bias, as in that case, one needs a lot of studies to reduce the effect size. Secondly, one can do an Egger's regression test, which tests whether the funnel plot is symmetrical. As mentioned before: a symmetrical funnel plot is a sign that there is no publication bias, as the effect size and sample size are not dependent. Thirdly, one can do the trim-and-fill method, which imputes data if the funnel plot is asymmetrical. |
||
The problem of publication bias is not trivial as it is suggested that 25% of meta-analyses in the psychological sciences may have suffered from publication bias.<ref name="Ferguson and Brannick"/> However, low power of existing tests and problems with the visual appearance of the funnel plot remain an issue, and estimates of publication bias may remain lower than what truly exists. |
The problem of publication bias is not trivial as it is suggested that 25% of meta-analyses in the psychological sciences may have suffered from publication bias.<ref name="Ferguson and Brannick"/> However, low power of existing tests and problems with the visual appearance of the funnel plot remain an issue, and estimates of publication bias may remain lower than what truly exists. |
||
Most discussions of publication bias focus on journal practices favoring publication of statistically significant findings. However, questionable research practices, such as reworking statistical models until significance is achieved, may also favor statistically significant findings in support of researchers' hypotheses.<ref name=Simmons>{{cite journal |vauthors=Simmons JP, Nelson LD, Simonsohn U | title = False-positive psychology: undisclosed flexibility in data collection and analysis allows presenting anything as significant | journal = |
Most discussions of publication bias focus on journal practices favoring publication of statistically significant findings. However, questionable research practices, such as reworking statistical models until significance is achieved, may also favor statistically significant findings in support of researchers' hypotheses.<ref name=Simmons>{{cite journal | vauthors = Simmons JP, Nelson LD, Simonsohn U | title = False-positive psychology: undisclosed flexibility in data collection and analysis allows presenting anything as significant | journal = Psychological Science | volume = 22 | issue = 11 | pages = 1359–1366 | date = November 2011 | pmid = 22006061 | doi = 10.1177/0956797611417632 | doi-access = free }}</ref><ref name=LeBel>{{Cite journal |year=2011 |author1=LeBel, E. |author2=Peters, K. |title=Fearing the future of empirical psychology: Bem's (2011) evidence of psi as a case study of deficiencies in modal research practice |journal=[[Review of General Psychology]] |volume=15 |issue=4 |pages=371–379 |url=http://publish.uwo.ca/~elebel/documents/l&p(2011,rgp).pdf |doi=10.1037/a0025172 |s2cid=51686730 |url-status=dead |archive-url=https://web.archive.org/web/20121124154834/http://publish.uwo.ca/~elebel/documents/l%26p%282011%2Crgp%29.pdf |archive-date=24 November 2012}}</ref> |
||
===Problems related to studies not reporting non-statistically significant effects=== |
===Problems related to studies not reporting non-statistically significant effects=== |
||
Studies often do not report the effects when they do not reach statistical significance{{citation needed|date=September 2018}}. For example, they may simply say that the groups did not show statistically significant differences, without reporting any other information (e.g. a statistic or p-value). Exclusion of these studies would lead to a situation similar to publication bias, but their inclusion (assuming null effects) would also bias the meta-analysis. MetaNSUE, a method created by [[Joaquim Radua]], has shown to allow researchers to include unbiasedly these studies.<ref>{{cite journal | |
Studies often do not report the effects when they do not reach statistical significance{{citation needed|date=September 2018}}. For example, they may simply say that the groups did not show statistically significant differences, without reporting any other information (e.g. a statistic or p-value). Exclusion of these studies would lead to a situation similar to publication bias, but their inclusion (assuming null effects) would also bias the meta-analysis. MetaNSUE, a method created by [[Joaquim Radua]], has shown to allow researchers to include unbiasedly these studies.<ref>{{cite journal | vauthors = Radua J, Schmidt A, Borgwardt S, Heinz A, Schlagenhauf F, McGuire P, Fusar-Poli P | title = Ventral Striatal Activation During Reward Processing in Psychosis: A Neurofunctional Meta-Analysis | journal = JAMA Psychiatry | volume = 72 | issue = 12 | pages = 1243–1251 | date = December 2015 | pmid = 26558708 | doi = 10.1001/jamapsychiatry.2015.2196 | doi-access = free }}</ref> Its steps are as follows: |
||
* [[Maximum likelihood estimation]] of the meta-analytic effect and the [[study heterogeneity|heterogeneity]] between studies. |
* [[Maximum likelihood estimation]] of the meta-analytic effect and the [[study heterogeneity|heterogeneity]] between studies. |
||
* [[Imputation (statistics)|Multiple imputation]] of the NSUEs adding noise to the estimate of the effect. |
* [[Imputation (statistics)|Multiple imputation]] of the NSUEs adding noise to the estimate of the effect. |
||
Line 151: | Line 151: | ||
===Problems arising from agenda-driven bias=== |
===Problems arising from agenda-driven bias=== |
||
The most severe fault in meta-analysis<ref>H. Sabhan</ref> often occurs when the person or persons doing the meta-analysis have an [[economic]], [[Social issues|social]], or [[political]] agenda such as the passage or defeat of [[legislation]]. People with these types of agendas may be more likely to abuse meta-analysis due to personal [[bias]]. For example, researchers favorable to the author's agenda are likely to have their studies [[Cherry picking (fallacy)|cherry-picked]] while those not favorable will be ignored or labeled as "not credible". In addition, the favored authors may themselves be biased or paid to produce results that support their overall political, social, or economic goals in ways such as selecting small favorable data sets and not incorporating larger unfavorable data sets. The influence of such biases on the results of a meta-analysis is possible because the methodology of meta-analysis is highly malleable.<ref name="Stegenga">{{cite journal | |
The most severe fault in meta-analysis<ref>H. Sabhan</ref> often occurs when the person or persons doing the meta-analysis have an [[economic]], [[Social issues|social]], or [[political]] agenda such as the passage or defeat of [[legislation]]. People with these types of agendas may be more likely to abuse meta-analysis due to personal [[bias]]. For example, researchers favorable to the author's agenda are likely to have their studies [[Cherry picking (fallacy)|cherry-picked]] while those not favorable will be ignored or labeled as "not credible". In addition, the favored authors may themselves be biased or paid to produce results that support their overall political, social, or economic goals in ways such as selecting small favorable data sets and not incorporating larger unfavorable data sets. The influence of such biases on the results of a meta-analysis is possible because the methodology of meta-analysis is highly malleable.<ref name="Stegenga">{{cite journal | vauthors = Stegenga J | title = Is meta-analysis the platinum standard of evidence? | journal = Studies in History and Philosophy of Biological and Biomedical Sciences | volume = 42 | issue = 4 | pages = 497–507 | date = December 2011 | pmid = 22035723 | doi = 10.1016/j.shpsc.2011.07.003 | author-link = Stegenga J }}</ref> |
||
A 2011 study done to disclose possible conflicts of interests in underlying research studies used for medical meta-analyses reviewed 29 meta-analyses and found that conflicts of interests in the studies underlying the meta-analyses were rarely disclosed. The 29 meta-analyses included 11 from general medicine journals, 15 from specialty medicine journals, and three from the [[Cochrane Database of Systematic Reviews]]. The 29 meta-analyses reviewed a total of 509 [[randomized controlled trials]] (RCTs). Of these, 318 RCTs reported funding sources, with 219 (69%) receiving funding from industry (i.e. one or more authors |
A 2011 study done to disclose possible conflicts of interests in underlying research studies used for medical meta-analyses reviewed 29 meta-analyses and found that conflicts of interests in the studies underlying the meta-analyses were rarely disclosed. The 29 meta-analyses included 11 from general medicine journals, 15 from specialty medicine journals, and three from the [[Cochrane Database of Systematic Reviews]]. The 29 meta-analyses reviewed a total of 509 [[randomized controlled trials]] (RCTs). Of these, 318 RCTs reported funding sources, with 219 (69%) receiving funding from industry (i.e. one or more authors |
||
having financial ties to the pharmaceutical industry). Of the 509 RCTs, 132 reported author conflict of interest disclosures, with 91 studies (69%) disclosing one or more authors having financial ties to industry. The information was, however, seldom reflected in the meta-analyses. Only two (7%) reported RCT funding sources and none reported RCT author-industry ties. The authors concluded "without acknowledgment of COI due to industry funding or author industry financial ties from RCTs included in meta-analyses, readers' understanding and appraisal of the evidence from the meta-analysis may be compromised."<ref>{{citation | title=Reporting of Conflicts of Interest in Meta-analyses of Trials of Pharmacological Treatments | journal = Journal of the American Medical Association | |
having financial ties to the pharmaceutical industry). Of the 509 RCTs, 132 reported author conflict of interest disclosures, with 91 studies (69%) disclosing one or more authors having financial ties to industry. The information was, however, seldom reflected in the meta-analyses. Only two (7%) reported RCT funding sources and none reported RCT author-industry ties. The authors concluded "without acknowledgment of COI due to industry funding or author industry financial ties from RCTs included in meta-analyses, readers' understanding and appraisal of the evidence from the meta-analysis may be compromised."<ref>{{citation | title=Reporting of Conflicts of Interest in Meta-analyses of Trials of Pharmacological Treatments | journal = Journal of the American Medical Association | vauthors = Roseman M, Milette K, Bero LA, Coyne JC, Lexchin J, Turner EH, Thombs BD | volume = 305 | issue = 10 | pages = 1008–1017 | year = 2011 | doi = 10.1001/jama.2011.257 | pmid = 21386079 | url = https://www.rug.nl/research/portal/en/publications/reporting-of-conflicts-of-interest-in-metaanalyses-of-trials-of-pharmacological-treatments(d4a95ee2-429f-45a4-a917-d794ee954797).html | hdl = 11370/d4a95ee2-429f-45a4-a917-d794ee954797 | hdl-access = free }}</ref> |
||
For example, in 1998, a US federal judge found that the United States [[Environmental Protection Agency]] had abused the meta-analysis process to produce a study claiming cancer risks to non-smokers from environmental tobacco smoke (ETS) with the intent to influence policy makers to pass smoke-free–workplace laws. The judge found that: |
For example, in 1998, a US federal judge found that the United States [[Environmental Protection Agency]] had abused the meta-analysis process to produce a study claiming cancer risks to non-smokers from environmental tobacco smoke (ETS) with the intent to influence policy makers to pass smoke-free–workplace laws. The judge found that: |
||
Line 163: | Line 163: | ||
===Weak inclusion standards lead to misleading conclusions=== |
===Weak inclusion standards lead to misleading conclusions=== |
||
Meta-analyses in education are often not restrictive enough in regards to the methodological quality of the studies they include. For example, studies that include small samples or researcher-made measures lead to inflated effect size estimates.<ref>{{Cite journal| |
Meta-analyses in education are often not restrictive enough in regards to the methodological quality of the studies they include. For example, studies that include small samples or researcher-made measures lead to inflated effect size estimates.<ref>{{Cite journal| vauthors = Cheung AC, Slavin RE |date=2016-06-01|title=How Methodological Features Affect Effect Sizes in Education |journal=Educational Researcher|language=en|volume=45|issue=5|pages=283–292|doi=10.3102/0013189X16656615|s2cid=148531062|issn=0013-189X}}</ref> However, this problem also troubles meta-analysis of clinical trials. The use of different quality assessment tools (QATs) lead to including different studies and obtaining conflicting estimates of average treatment effects<ref>{{cite journal | vauthors = Jüni P, Witschi A, Bloch R, Egger M | title = The hazards of scoring the quality of clinical trials for meta-analysis | journal = Jama | volume = 282 | issue = 11 | pages = 1054–1060 | date = September 1999 | pmid = 10493204 | doi = 10.1001/jama.282.11.1054 }}</ref><ref>{{cite journal | vauthors = Armijo-Olivo S, Fuentes J, Ospina M, Saltaji H, Hartling L | title = Inconsistency in the items included in tools used in general health research and physical therapy to evaluate the methodological quality of randomized controlled trials: a descriptive analysis | journal = BMC Medical Research Methodology | volume = 13 | issue = 1 | pages = 116 | date = September 2013 | pmid = 24044807 | pmc = 3848693 | doi = 10.1186/1471-2288-13-116 }}</ref>. |
||
==Applications in modern science== |
==Applications in modern science== |
||
Modern statistical meta-analysis does more than just combine the effect sizes of a set of studies using a weighted average. It can test if the outcomes of studies show more variation than the variation that is expected because of the sampling of different numbers of research participants. Additionally, study characteristics such as measurement instrument used, population sampled, or aspects of the studies' design can be coded and used to reduce variance of the estimator (see statistical models above). Thus some methodological weaknesses in studies can be corrected statistically. Other uses of meta-analytic methods include the development and validation of clinical prediction models, where meta-analysis may be used to combine individual participant data from different research centers and to assess the model's generalisability,<ref>{{cite journal | |
Modern statistical meta-analysis does more than just combine the effect sizes of a set of studies using a weighted average. It can test if the outcomes of studies show more variation than the variation that is expected because of the sampling of different numbers of research participants. Additionally, study characteristics such as measurement instrument used, population sampled, or aspects of the studies' design can be coded and used to reduce variance of the estimator (see statistical models above). Thus some methodological weaknesses in studies can be corrected statistically. Other uses of meta-analytic methods include the development and validation of clinical prediction models, where meta-analysis may be used to combine individual participant data from different research centers and to assess the model's generalisability,<ref>{{cite journal | vauthors = Debray TP, Riley RD, Rovers MM, Reitsma JB, Moons KG | title = Individual participant data (IPD) meta-analyses of diagnostic and prognostic modeling studies: guidance on their use | journal = PLoS Medicine | volume = 12 | issue = 10 | pages = e1001886 | date = October 2015 | pmid = 26461078 | pmc = 4603958 | doi = 10.1371/journal.pmed.1001886 }}</ref><ref>{{cite journal | vauthors = Debray TP, Moons KG, Ahmed I, Koffijberg H, Riley RD | title = A framework for developing, implementing, and evaluating clinical prediction models in an individual participant data meta-analysis | journal = Statistics in Medicine | volume = 32 | issue = 18 | pages = 3158–3180 | date = August 2013 | pmid = 23307585 | doi = 10.1002/sim.5732 }}</ref> or even to aggregate existing prediction models.<ref>{{cite journal | vauthors = Debray TP, Koffijberg H, Vergouwe Y, Moons KG, Steyerberg EW | title = Aggregating published prediction models with individual participant data: a comparison of different approaches | journal = Statistics in Medicine | volume = 31 | issue = 23 | pages = 2697–2712 | date = October 2012 | pmid = 22733546 | doi = 10.1002/sim.5412 }}</ref> |
||
Meta-analysis can be done with [[single-subject design]] as well as group research designs. This is important because much research has been done with [[single-subject research]] designs. Considerable dispute exists for the most appropriate meta-analytic technique for single subject research.<ref>{{cite journal |vauthors=Van den Noortgate W, Onghena P | year = 2007 | title = Aggregating Single-Case Results | journal = The Behavior Analyst Today | volume = 8 | issue = 2 | pages = 196–209 | url = https://www.questia.com/read/1G1-170115042/the-aggregation-of-single-case-results-using-hierarchical | doi=10.1037/h0100613}}</ref> |
Meta-analysis can be done with [[single-subject design]] as well as group research designs. This is important because much research has been done with [[single-subject research]] designs. Considerable dispute exists for the most appropriate meta-analytic technique for single subject research.<ref>{{cite journal |vauthors=Van den Noortgate W, Onghena P | year = 2007 | title = Aggregating Single-Case Results | journal = The Behavior Analyst Today | volume = 8 | issue = 2 | pages = 196–209 | url = https://www.questia.com/read/1G1-170115042/the-aggregation-of-single-case-results-using-hierarchical | doi=10.1037/h0100613}}</ref> |
||
Line 173: | Line 173: | ||
Meta-analysis leads to a shift of emphasis from single studies to multiple studies. It emphasizes the practical importance of the effect size instead of the statistical significance of individual studies. This shift in thinking has been termed "meta-analytic thinking". The results of a meta-analysis are often shown in a [[forest plot]]. |
Meta-analysis leads to a shift of emphasis from single studies to multiple studies. It emphasizes the practical importance of the effect size instead of the statistical significance of individual studies. This shift in thinking has been termed "meta-analytic thinking". The results of a meta-analysis are often shown in a [[forest plot]]. |
||
Results from studies are combined using different approaches. One approach frequently used in meta-analysis in health care research is termed '[[Inverse-variance weighting|inverse variance method]]'. The average [[effect size]] across all studies is computed as a ''weighted mean'', whereby the weights are equal to the inverse variance of each study's effect estimator. Larger studies and studies with less random variation are given greater weight than smaller studies. Other common approaches include the [[Cochran–Mantel–Haenszel statistics|Mantel–Haenszel method]]<ref>{{cite journal |vauthors=Mantel N, Haenszel W | title = Statistical aspects of the analysis of data from |
Results from studies are combined using different approaches. One approach frequently used in meta-analysis in health care research is termed '[[Inverse-variance weighting|inverse variance method]]'. The average [[effect size]] across all studies is computed as a ''weighted mean'', whereby the weights are equal to the inverse variance of each study's effect estimator. Larger studies and studies with less random variation are given greater weight than smaller studies. Other common approaches include the [[Cochran–Mantel–Haenszel statistics|Mantel–Haenszel method]]<ref>{{cite journal | vauthors = Mantel N, Haenszel W | title = Statistical aspects of the analysis of data from retrospective studies of disease | journal = Journal of the National Cancer Institute | volume = 22 | issue = 4 | pages = 719–748 | date = April 1959 | pmid = 13655060 | doi = 10.1093/jnci/22.4.719 | s2cid = 17698270 }}</ref> |
||
and the [[Richard Peto|Peto method]].<ref>{{cite web|title=9.4.4.2 Peto odds ratio method|url=http://handbook.cochrane.org/chapter_9/9_4_4_2_peto_odds_ratio_method.htm|publisher=Cochrane Handbook for Systematic Reviews of Interventions v 5.1.0|date=March 2011}}</ref> |
and the [[Richard Peto|Peto method]].<ref>{{cite web|title=9.4.4.2 Peto odds ratio method|url=http://handbook.cochrane.org/chapter_9/9_4_4_2_peto_odds_ratio_method.htm|publisher=Cochrane Handbook for Systematic Reviews of Interventions v 5.1.0|date=March 2011}}</ref> |
||
[[Seed-based d mapping]] (formerly signed differential mapping, SDM) is a statistical technique for meta-analyzing studies on differences in brain activity or structure which used neuroimaging techniques such as fMRI, VBM or PET. |
[[Seed-based d mapping]] (formerly signed differential mapping, SDM) is a statistical technique for meta-analyzing studies on differences in brain activity or structure which used neuroimaging techniques such as fMRI, VBM or PET. |
||
Different high throughput techniques such as [[DNA microarray|microarrays]] have been used to understand [[Gene expression]]. [[MicroRNA]] expression profiles have been used to identify differentially expressed microRNAs in particular cell or tissue type or disease conditions or to check the effect of a treatment. A meta-analysis of such expression profiles was performed to derive novel conclusions and to validate the known findings.<ref>{{cite journal |vauthors=Bargaje R, Hariharan M, Scaria V, Pillai B | title = Consensus miRNA expression profiles derived from interplatform normalization of microarray data | journal = |
Different high throughput techniques such as [[DNA microarray|microarrays]] have been used to understand [[Gene expression]]. [[MicroRNA]] expression profiles have been used to identify differentially expressed microRNAs in particular cell or tissue type or disease conditions or to check the effect of a treatment. A meta-analysis of such expression profiles was performed to derive novel conclusions and to validate the known findings.<ref>{{cite journal | vauthors = Bargaje R, Hariharan M, Scaria V, Pillai B | title = Consensus miRNA expression profiles derived from interplatform normalization of microarray data | journal = Rna | volume = 16 | issue = 1 | pages = 16–25 | date = January 2010 | pmid = 19948767 | pmc = 2802026 | doi = 10.1261/rna.1688110 }}</ref> |
||
==See also== |
== See also == |
||
{{Portal|Mathematics}} |
{{Portal|Mathematics}} |
||
* [[Estimation statistics]] |
* [[Estimation statistics]] |
||
Line 193: | Line 193: | ||
* [[Data aggregation]] |
* [[Data aggregation]] |
||
==References== |
== References == |
||
{{Reflist|30em|refs=<ref name=Zhang2020>{{cite journal | vauthors = Zhang H, Deng L, Schiffman M, Qin J, Yu K | title = Generalized integration model for improved statistical inference by leveraging external summary data | journal = Biometrika | date = 2020 | volume = 107 | issue = 3 | pages = 689–703 | doi = 10.1093/biomet/asaa014 }}</ref> |
{{Reflist|30em|refs=<ref name=Zhang2020>{{cite journal | vauthors = Zhang H, Deng L, Schiffman M, Qin J, Yu K | title = Generalized integration model for improved statistical inference by leveraging external summary data | journal = Biometrika | date = 2020 | volume = 107 | issue = 3 | pages = 689–703 | doi = 10.1093/biomet/asaa014 }}</ref> |
||
}} |
}} |
||
==Further reading== |
== Further reading == |
||
{{refbegin| |
{{refbegin|30em}} |
||
* Cooper |
* {{cite book | veditors = Cooper H, Hedges LV |title=The Handbook of Research Synthesis |date=1994 |publisher=Russell Sage Foundation |location=New York |isbn=978-0-87154-226-7}} |
||
* Cornell, |
* {{cite book | vauthors = Cornell JE, Mulrow CD | pages = 285–323 | chapter = Meta-analysis | veditors = Mellenbergh GJ | editor-link1 = Gideon J. Mellenbergh |title=Research methodology in the life, behavioural, and social sciences |date=1999 |publisher=SAGE |location=London |isbn=978-0-7619-5883-3}} |
||
* {{cite journal | |
* {{cite journal | vauthors = Normand SL | title = Meta-analysis: formulating, evaluating, combining, and reporting | journal = Statistics in Medicine | volume = 18 | issue = 3 | pages = 321–359 | date = February 1999 | pmid = 10070677 | doi = 10.1002/(SICI)1097-0258(19990215)18:3<321::AID-SIM28>3.0.CO;2-P | author-link = Sharon-Lise Normand }} |
||
* Sutton, A.J., Jones, D.R., Abrams, K.R., Sheldon, T.A., & Song, F. (2000). ''Methods for Meta-analysis in Medical Research''. London: John Wiley. {{ISBN|0-471-49066-0}} |
* Sutton, A.J., Jones, D.R., Abrams, K.R., Sheldon, T.A., & Song, F. (2000). ''Methods for Meta-analysis in Medical Research''. London: John Wiley. {{ISBN|0-471-49066-0}} |
||
* Higgins JPT, Green S (editors). Cochrane Handbook for Systematic Reviews of Interventions Version 5.0.1 [updated September 2008]. The Cochrane Collaboration, 2008. Available from www.cochrane-handbook.org |
* Higgins JPT, Green S (editors). Cochrane Handbook for Systematic Reviews of Interventions Version 5.0.1 [updated September 2008]. The Cochrane Collaboration, 2008. Available from www.cochrane-handbook.org |
||
* {{cite journal | |
* {{cite journal | vauthors = Thompson SG, Pocock SJ | title = Can meta-analyses be trusted? | journal = Lancet | volume = 338 | issue = 8775 | pages = 1127–1130 | date = November 1991 | pmid = 1682553 | doi = 10.1016/0140-6736(91)91975-Z | url = http://tobaccodocuments.org/pm/2047231315-1318.pdf | access-date = 17 June 2011 | url-status = dead | s2cid = 29743240 | archive-url = https://web.archive.org/web/20111122083418/http://tobaccodocuments.org/pm/2047231315-1318.pdf | archive-date = 22 November 2011 }}. Explores two contrasting views: does meta-analysis provide "objective, quantitative methods for combining evidence from separate but similar studies" or merely "statistical tricks which make unjustified assumptions in producing oversimplified generalisations out of a complex of disparate studies"? |
||
* Wilson, D. B., & Lipsey, M. W. (2001). ''Practical meta-analysis''. Thousand Oaks: Sage publications. {{ISBN|0-7619-2168-0}} |
* Wilson, D. B., & Lipsey, M. W. (2001). ''Practical meta-analysis''. Thousand Oaks: Sage publications. {{ISBN|0-7619-2168-0}} |
||
* O'Rourke, K. (2007) [https://web.archive.org/web/20111102182723/http://andrewgelman.com/movabletype/mlm/JustHistory.pdf Just the history from the combining of information: investigating and synthesizing what is possibly common in clinical observations or studies via likelihood.] Oxford: University of Oxford, Department of Statistics. Gives technical background material and details on the "An historical perspective on meta-analysis" paper cited in the references. |
* O'Rourke, K. (2007) [https://web.archive.org/web/20111102182723/http://andrewgelman.com/movabletype/mlm/JustHistory.pdf Just the history from the combining of information: investigating and synthesizing what is possibly common in clinical observations or studies via likelihood.] Oxford: University of Oxford, Department of Statistics. Gives technical background material and details on the "An historical perspective on meta-analysis" paper cited in the references. |
||
Line 210: | Line 210: | ||
* Ellis, Paul D. (2010). ''The Essential Guide to Effect Sizes: An Introduction to Statistical Power, Meta-Analysis and the Interpretation of Research Results''. United Kingdom: Cambridge University Press. {{ISBN|0-521-14246-6}} |
* Ellis, Paul D. (2010). ''The Essential Guide to Effect Sizes: An Introduction to Statistical Power, Meta-Analysis and the Interpretation of Research Results''. United Kingdom: Cambridge University Press. {{ISBN|0-521-14246-6}} |
||
* Bonett, DG (2020) ''Point-biserial correlation: Confidence intervals, hypothesis testing, meta-analysis, and sample size planning.'' British Journal of Mathematical and Statistical Psychology 73. doi: 10.1111/bmsp.12189 |
* Bonett, DG (2020) ''Point-biserial correlation: Confidence intervals, hypothesis testing, meta-analysis, and sample size planning.'' British Journal of Mathematical and Statistical Psychology 73. doi: 10.1111/bmsp.12189 |
||
* {{cite journal |vauthors=Bonett DG, Price RM | title = Varying coefficient meta-analysis methods for odds ratios and risk ratios | journal = |
* {{cite journal | vauthors = Bonett DG, Price RM | title = Varying coefficient meta-analysis methods for odds ratios and risk ratios | journal = Psychological Methods | volume = 20 | issue = 3 | pages = 394–406 | date = September 2015 | pmid = 25751513 | doi = 10.1037/met0000032 }} |
||
* {{cite journal |vauthors=Bonett DG, Price RM | title = Meta-analysis methods for risk differences | journal = |
* {{cite journal | vauthors = Bonett DG, Price RM | title = Meta-analysis methods for risk differences | journal = The British Journal of Mathematical and Statistical Psychology | volume = 67 | issue = 3 | pages = 371–387 | date = November 2014 | pmid = 23962020 | doi = 10.1111/bmsp.12024 }} |
||
* {{cite journal | |
* {{cite journal | vauthors = Bonett DG | title = Varying coefficient meta-analytic methods for alpha reliability | journal = Psychological Methods | volume = 15 | issue = 4 | pages = 368–385 | date = December 2010 | pmid = 20853952 | doi = 10.1037/a0020142 | s2cid = 207710319 }} |
||
* {{cite journal | |
* {{cite journal | vauthors = Bonett DG | title = Meta-analytic interval estimation for standardized and unstandardized mean differences | journal = Psychological Methods | volume = 14 | issue = 3 | pages = 225–238 | date = September 2009 | pmid = 19719359 | doi = 10.1037/a0016619 }} |
||
* {{cite journal | |
* {{cite journal | vauthors = Bonett DG | title = Meta-analytic interval estimation for bivariate correlations | journal = Psychological Methods | volume = 13 | issue = 3 | pages = 173–181 | date = September 2008 | pmid = 18778150 | doi = 10.1037/a0012868 | s2cid = 5690835 }} |
||
{{refend}} |
{{refend}} |
||
==External links== |
== External links == |
||
{{wikiversity}} |
{{wikiversity}} |
||
* [http://handbook-5-1.cochrane.org/ Cochrane Handbook for Systematic Reviews of Interventions] |
* [http://handbook-5-1.cochrane.org/ Cochrane Handbook for Systematic Reviews of Interventions] |
Revision as of 06:42, 23 December 2021
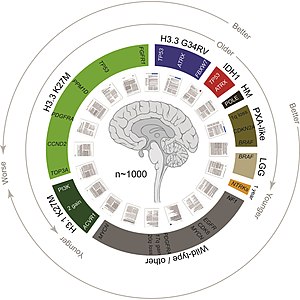
Part of a series on |
Research |
---|
Philosophy portal |
A meta-analysis is a statistical analysis that combines the results of multiple scientific studies. Meta-analyses can be performed when there are multiple scientific studies addressing the same question, with each individual study reporting measurements that are expected to have some degree of error. The aim then is to use approaches from statistics to derive a pooled estimate closest to the unknown common truth based on how this error is perceived. Meta-analytic results are considered the most trustworthy source of evidence by the evidence-based medicine literature[1][2].
Not only can meta-analyses provide an estimate of the unknown effect size, it also has the capacity to contrast results from different studies and identify patterns among study results, sources of disagreement among those results, or other interesting relationships that may come to light with multiple studies.[3]
However, there are some methodological problems with meta-analysis. If individual studies are systematically biased due to questionable research practices (e.g., p-hacking, data peeking, dropping studies) or the publication bias at the journal level, the meta-analytic estimate of the overall treatment effect may not reflect the actual efficacy of a treatment[4][5]. Meta-analysis has also been criticized for averaging differences among heterogeneous studies because these differences could potentially inform clinical decisions[6]. For example, if there are two groups of patients experiencing different treatment effects studies in two RCTs reporting conflicting results, the meta-analytic average is representative of neither group, similarly to averaging the weight of apples and oranges, which is neither accurate for apples nor oranges[7]. In performing a meta-analysis, an investigator must make choices which can affect the results, including deciding how to search for studies, selecting studies based on a set of objective criteria, dealing with incomplete data, analyzing the data, and accounting for or choosing not to account for publication bias.[8] This makes meta-analysis malleable in the sense that these methodological choices made in completing a meta-analysis are not determined but may affect the results[9]. For instance, a meta-analysis of the same RCTs on the efficacy of local anesthesia and meta-regression of observational studies on the effects of meat consumption with, respectively, seven and eight meta-analytic techniques reported different p values of obtained average ATEs[10]. For example, Wanous and colleagues examined four pairs of meta-analyses on the four topics of (a) job performance and satisfaction relationship, (b) realistic job previews, (c) correlates of role conflict and ambiguity, and (d) the job satisfaction and absenteeism relationship, and illustrated how various judgement calls made by the researchers produced different results.[11]
Meta-analyses are often, but not always, important components of a systematic review procedure. For instance, a meta-analysis may be conducted on several clinical trials of a medical treatment, in an effort to obtain a better understanding of how well the treatment works. Here it is convenient to follow the terminology used by the Cochrane Collaboration,[12] and use "meta-analysis" to refer to statistical methods of combining evidence, leaving other aspects of 'research synthesis' or 'evidence synthesis', such as combining information from qualitative studies, for the more general context of systematic reviews. A meta-analysis is a secondary source.[13][14] In addition, meta-analysis may also be applied to a single study in cases where there are many cohorts which have not gone through identical selection criteria or to which the same investigational methologies have not been applied to all in the same manner or under the same exacting conditions. Under these circumstances each cohort is treated as an individual study and meta-analysis is used to draw study-wide conclusions.[15]
History
The historical roots of meta-analysis can be traced back to 17th century studies of astronomy,[16] while a paper published in 1904 by the statistician Karl Pearson in the British Medical Journal[17] which collated data from several studies of typhoid inoculation is seen as the first time a meta-analytic approach was used to aggregate the outcomes of multiple clinical studies.[18][19] The first meta-analysis of all conceptually identical experiments concerning a particular research issue, and conducted by independent researchers, has been identified as the 1940 book-length publication Extrasensory Perception After Sixty Years, authored by Duke University psychologists J. G. Pratt, J. B. Rhine, and associates.[20] This encompassed a review of 145 reports on ESP experiments published from 1882 to 1939, and included an estimate of the influence of unpublished papers on the overall effect (the file-drawer problem). The term "meta-analysis" was coined in 1976 by the statistician Gene V. Glass,[21] who stated "my major interest currently is in what we have come to call ...the meta-analysis of research. The term is a bit grand, but it is precise and apt ... Meta-analysis refers to the analysis of analyses". Although this led to him being widely recognized as the modern founder of the method, the methodology behind what he termed "meta-analysis" predates his work by several decades.[22][23] The statistical theory surrounding meta-analysis was greatly advanced by the work of Nambury S. Raju, Larry V. Hedges, Harris Cooper, Ingram Olkin, John E. Hunter, Jacob Cohen, Thomas C. Chalmers, Robert Rosenthal, Frank L. Schmidt, John E. Hunter, and Douglas G. Claurett.[clarification needed] In 1992, meta-analysis was first applied to ecological questions[24] by Jessica Gurevitch who used meta-analysis to study competition in field experiments.[25][26]
Steps in a meta-analysis
A meta-analysis is usually preceded by a systematic review, as this allows identification and critical appraisal of all the relevant evidence (thereby limiting the risk of bias in summary estimates). The general steps are then as follows:
- Formulation of the research question, e.g. using the PICO model (Population, Intervention, Comparison, Outcome).
- Search of literature
- Selection of studies ('incorporation criteria')
- Based on quality criteria, e.g. the requirement of randomization and blinding in a clinical trial
- Selection of specific studies on a well-specified subject, e.g. the treatment of breast cancer.
- Decide whether unpublished studies are included to avoid publication bias (file drawer problem)
- Decide which dependent variables or summary measures are allowed. For instance, when considering a meta-analysis of published (aggregate) data:
- Differences (discrete data)
- Means (continuous data)
- Hedges' g is a popular summary measure for continuous data that is standardized in order to eliminate scale differences, but it incorporates an index of variation between groups:
- in which is the treatment mean, is the control mean, the pooled variance.
- Selection of a meta-analysis model, e.g. fixed effect or random effects meta-analysis.
- Examine sources of between-study heterogeneity, e.g. using subgroup analysis or meta-regression.
Formal guidance for the conduct and reporting of meta-analyses is provided by the Cochrane Handbook.
For reporting guidelines, see the Preferred Reporting Items for Systematic Reviews and Meta-Analyses (PRISMA) statement.[27]
Methods and assumptions
Approaches
In general, two types of evidence can be distinguished when performing a meta-analysis: individual participant data (IPD), and aggregate data (AD). The aggregate data can be direct or indirect.
AD is more commonly available (e.g. from the literature) and typically represents summary estimates such as odds ratios or relative risks. This can be directly synthesized across conceptually similar studies using several approaches (see below). On the other hand, indirect aggregate data measures the effect of two treatments that were each compared against a similar control group in a meta-analysis. For example, if treatment A and treatment B were directly compared vs placebo in separate meta-analyses, we can use these two pooled results to get an estimate of the effects of A vs B in an indirect comparison as effect A vs Placebo minus effect B vs Placebo.
IPD evidence represents raw data as collected by the study centers. This distinction has raised the need for different meta-analytic methods when evidence synthesis is desired, and has led to the development of one-stage and two-stage methods.[28] In one-stage methods the IPD from all studies are modeled simultaneously whilst accounting for the clustering of participants within studies. Two-stage methods first compute summary statistics for AD from each study and then calculate overall statistics as a weighted average of the study statistics. By reducing IPD to AD, two-stage methods can also be applied when IPD is available; this makes them an appealing choice when performing a meta-analysis. Although it is conventionally believed that one-stage and two-stage methods yield similar results, recent studies have shown that they may occasionally lead to different conclusions.[29][30]
Statistical models for aggregate data
Direct evidence: Models incorporating study effects only
Fixed effects model
The fixed effect model provides a weighted average of a series of study estimates. The inverse of the estimates' variance is commonly used as study weight, so that larger studies tend to contribute more than smaller studies to the weighted average. Consequently, when studies within a meta-analysis are dominated by a very large study, the findings from smaller studies are practically ignored.[31] Most importantly, the fixed effects model assumes that all included studies investigate the same population, use the same variable and outcome definitions, etc. This assumption is typically unrealistic as research is often prone to several sources of heterogeneity; e.g. treatment effects may differ according to locale, dosage levels, study conditions, ...
Random effects model
A common model used to synthesize heterogeneous research is the random effects model of meta-analysis. This is simply the weighted average of the effect sizes of a group of studies. The weight that is applied in this process of weighted averaging with a random effects meta-analysis is achieved in two steps:[32]
- Step 1: Inverse variance weighting
- Step 2: Un-weighting of this inverse variance weighting by applying a random effects variance component (REVC) that is simply derived from the extent of variability of the effect sizes of the underlying studies.
This means that the greater this variability in effect sizes (otherwise known as heterogeneity), the greater the un-weighting and this can reach a point when the random effects meta-analysis result becomes simply the un-weighted average effect size across the studies. At the other extreme, when all effect sizes are similar (or variability does not exceed sampling error), no REVC is applied and the random effects meta-analysis defaults to simply a fixed effect meta-analysis (only inverse variance weighting).
The extent of this reversal is solely dependent on two factors:[33]
- Heterogeneity of precision
- Heterogeneity of effect size
Since neither of these factors automatically indicates a faulty larger study or more reliable smaller studies, the re-distribution of weights under this model will not bear a relationship to what these studies actually might offer. Indeed, it has been demonstrated that redistribution of weights is simply in one direction from larger to smaller studies as heterogeneity increases until eventually all studies have equal weight and no more redistribution is possible.[33] Another issue with the random effects model is that the most commonly used confidence intervals generally do not retain their coverage probability above the specified nominal level and thus substantially underestimate the statistical error and are potentially overconfident in their conclusions.[34][35] Several fixes have been suggested[36][37] but the debate continues on.[35][38] A further concern is that the average treatment effect can sometimes be even less conservative compared to the fixed effect model[39] and therefore misleading in practice. One interpretational fix that has been suggested is to create a prediction interval around the random effects estimate to portray the range of possible effects in practice.[40] However, an assumption behind the calculation of such a prediction interval is that trials are considered more or less homogeneous entities and that included patient populations and comparator treatments should be considered exchangeable[41] and this is usually unattainable in practice.
The most widely used method to estimate between studies variance (REVC) is the DerSimonian-Laird (DL) approach.[42] Several advanced iterative (and computationally expensive) techniques for computing the between studies variance exist (such as maximum likelihood, profile likelihood and restricted maximum likelihood methods) and random effects models using these methods can be run in Stata with the metaan command.[43] The metaan command must be distinguished from the classic metan (single "a") command in Stata that uses the DL estimator. These advanced methods have also been implemented in a free and easy to use Microsoft Excel add-on, MetaEasy.[44][45] However, a comparison between these advanced methods and the DL method of computing the between studies variance demonstrated that there is little to gain and DL is quite adequate in most scenarios.[46][47]
However, most meta-analyses include between 2 and 4 studies and such a sample is more often than not inadequate to accurately estimate heterogeneity. Thus it appears that in small meta-analyses, an incorrect zero between study variance estimate is obtained, leading to a false homogeneity assumption. Overall, it appears that heterogeneity is being consistently underestimated in meta-analyses and sensitivity analyses in which high heterogeneity levels are assumed could be informative.[48] These random effects models and software packages mentioned above relate to study-aggregate meta-analyses and researchers wishing to conduct individual patient data (IPD) meta-analyses need to consider mixed-effects modelling approaches.[49]
IVhet model
Doi & Barendregt working in collaboration with Khan, Thalib and Williams (from the University of Queensland, University of Southern Queensland and Kuwait University), have created an inverse variance quasi likelihood based alternative (IVhet) to the random effects (RE) model for which details are available online.[50] This was incorporated into MetaXL version 2.0,[51] a free Microsoft excel add-in for meta-analysis produced by Epigear International Pty Ltd, and made available on 5 April 2014. The authors state that a clear advantage of this model is that it resolves the two main problems of the random effects model. The first advantage of the IVhet model is that coverage remains at the nominal (usually 95%) level for the confidence interval unlike the random effects model which drops in coverage with increasing heterogeneity.[34][35] The second advantage is that the IVhet model maintains the inverse variance weights of individual studies, unlike the RE model which gives small studies more weight (and therefore larger studies less) with increasing heterogeneity. When heterogeneity becomes large, the individual study weights under the RE model become equal and thus the RE model returns an arithmetic mean rather than a weighted average. This side-effect of the RE model does not occur with the IVhet model which thus differs from the RE model estimate in two perspectives:[50] Pooled estimates will favor larger trials (as opposed to penalizing larger trials in the RE model) and will have a confidence interval that remains within the nominal coverage under uncertainty (heterogeneity). Doi & Barendregt suggest that while the RE model provides an alternative method of pooling the study data, their simulation results[52] demonstrate that using a more specified probability model with untenable assumptions, as with the RE model, does not necessarily provide better results. The latter study also reports that the IVhet model resolves the problems related to underestimation of the statistical error, poor coverage of the confidence interval and increased MSE seen with the random effects model and the authors conclude that researchers should henceforth abandon use of the random effects model in meta-analysis. While their data is compelling, the ramifications (in terms of the magnitude of spuriously positive results within the Cochrane database) are huge and thus accepting this conclusion requires careful independent confirmation. The availability of a free software (MetaXL)[51] that runs the IVhet model (and all other models for comparison) facilitates this for the research community.
Direct evidence: Models incorporating additional information
Quality effects model
Doi and Thalib originally introduced the quality effects model.[53] They[54] introduced a new approach to adjustment for inter-study variability by incorporating the contribution of variance due to a relevant component (quality) in addition to the contribution of variance due to random error that is used in any fixed effects meta-analysis model to generate weights for each study. The strength of the quality effects meta-analysis is that it allows available methodological evidence to be used over subjective random effects, and thereby helps to close the damaging gap which has opened up between methodology and statistics in clinical research. To do this a synthetic bias variance is computed based on quality information to adjust inverse variance weights and the quality adjusted weight of the ith study is introduced.[53] These adjusted weights are then used in meta-analysis. In other words, if study i is of good quality and other studies are of poor quality, a proportion of their quality adjusted weights is mathematically redistributed to study i giving it more weight towards the overall effect size. As studies become increasingly similar in terms of quality, re-distribution becomes progressively less and ceases when all studies are of equal quality (in the case of equal quality, the quality effects model defaults to the IVhet model – see previous section). A recent evaluation of the quality effects model (with some updates) demonstrates that despite the subjectivity of quality assessment, the performance (MSE and true variance under simulation) is superior to that achievable with the random effects model.[55][56] This model thus replaces the untenable interpretations that abound in the literature and a software is available to explore this method further.[51]
Indirect evidence: Network meta-analysis methods

Indirect comparison meta-analysis methods (also called network meta-analyses, in particular when multiple treatments are assessed simultaneously) generally use two main methodologies. First, is the Bucher method[57] which is a single or repeated comparison of a closed loop of three-treatments such that one of them is common to the two studies and forms the node where the loop begins and ends. Therefore, multiple two-by-two comparisons (3-treatment loops) are needed to compare multiple treatments. This methodology requires that trials with more than two arms have two arms only selected as independent pair-wise comparisons are required. The alternative methodology uses complex statistical modelling to include the multiple arm trials and comparisons simultaneously between all competing treatments. These have been executed using Bayesian methods, mixed linear models and meta-regression approaches.[citation needed]
Bayesian framework
Specifying a Bayesian network meta-analysis model involves writing a directed acyclic graph (DAG) model for general-purpose Markov chain Monte Carlo (MCMC) software such as WinBUGS.[58] In addition, prior distributions have to be specified for a number of the parameters, and the data have to be supplied in a specific format.[58] Together, the DAG, priors, and data form a Bayesian hierarchical model. To complicate matters further, because of the nature of MCMC estimation, overdispersed starting values have to be chosen for a number of independent chains so that convergence can be assessed.[59] Currently, there is no software that automatically generates such models, although there are some tools to aid in the process. The complexity of the Bayesian approach has limited usage of this methodology. Methodology for automation of this method has been suggested[60] but requires that arm-level outcome data are available, and this is usually unavailable. Great claims are sometimes made for the inherent ability of the Bayesian framework to handle network meta-analysis and its greater flexibility. However, this choice of implementation of framework for inference, Bayesian or frequentist, may be less important than other choices regarding the modeling of effects[61] (see discussion on models above).
Frequentist multivariate framework
On the other hand, the frequentist multivariate methods involve approximations and assumptions that are not stated explicitly or verified when the methods are applied (see discussion on meta-analysis models above). For example, the mvmeta package for Stata enables network meta-analysis in a frequentist framework.[62] However, if there is no common comparator in the network, then this has to be handled by augmenting the dataset with fictional arms with high variance, which is not very objective and requires a decision as to what constitutes a sufficiently high variance.[63] The other issue is use of the random effects model in both this frequentist framework and the Bayesian framework. Senn advises analysts to be cautious about interpreting the 'random effects' analysis since only one random effect is allowed for but one could envisage many.[61] Senn goes on to say that it is rather naıve, even in the case where only two treatments are being compared to assume that random-effects analysis accounts for all uncertainty about the way effects can vary from trial to trial. Newer models of meta-analysis such as those discussed above would certainly help alleviate this situation and have been implemented in the next framework.
Generalized pairwise modelling framework
An approach that has been tried since the late 1990s is the implementation of the multiple three-treatment closed-loop analysis. This has not been popular because the process rapidly becomes overwhelming as network complexity increases. Development in this area was then abandoned in favor of the Bayesian and multivariate frequentist methods which emerged as alternatives. Very recently, automation of the three-treatment closed loop method has been developed for complex networks by some researchers[50] as a way to make this methodology available to the mainstream research community. This proposal does restrict each trial to two interventions, but also introduces a workaround for multiple arm trials: a different fixed control node can be selected in different runs. It also utilizes robust meta-analysis methods so that many of the problems highlighted above are avoided. Further research around this framework is required to determine if this is indeed superior to the Bayesian or multivariate frequentist frameworks. Researchers willing to try this out have access to this framework through a free software.[51]
Tailored meta-analysis
Another form of additional information comes from the intended setting. If the target setting for applying the meta-analysis results is known then it may be possible to use data from the setting to tailor the results thus producing a 'tailored meta-analysis'.,[64][65] This has been used in test accuracy meta-analyses, where empirical knowledge of the test positive rate and the prevalence have been used to derive a region in Receiver Operating Characteristic (ROC) space known as an 'applicable region'. Studies are then selected for the target setting based on comparison with this region and aggregated to produce a summary estimate which is tailored to the target setting.
Aggregating IPD and AD
Meta-analysis can also be applied to combine IPD and AD. This is convenient when the researchers who conduct the analysis have their own raw data while collecting aggregate or summary data from the literature. The generalized integration model (GIM)[66] is a generalization of the meta-analysis. It allows that the model fitted on the individual participant data (IPD) is different from the ones used to compute the aggregate data (AD). GIM can be viewed as a model calibration method for integrating information with more flexibility.
Validation of meta-analysis results
The meta-analysis estimate represents a weighted average across studies and when there is heterogeneity this may result in the summary estimate not being representative of individual studies. Qualitative appraisal of the primary studies using established tools can uncover potential biases,[67][68] but does not quantify the aggregate effect of these biases on the summary estimate. Although the meta-analysis result could be compared with an independent prospective primary study, such external validation is often impractical. This has led to the development of methods that exploit a form of leave-one-out cross validation, sometimes referred to as internal-external cross validation (IOCV).[69] Here each of the k included studies in turn is omitted and compared with the summary estimate derived from aggregating the remaining k- 1 studies. A general validation statistic, Vn based on IOCV has been developed to measure the statistical validity of meta-analysis results.[70] For test accuracy and prediction, particularly when there are multivariate effects, other approaches which seek to estimate the prediction error have also been proposed.[71]
Challenges
A meta-analysis of several small studies does not always predict the results of a single large study.[72] Some have argued that a weakness of the method is that sources of bias are not controlled by the method: a good meta-analysis cannot correct for poor design or bias in the original studies.[73] This would mean that only methodologically sound studies should be included in a meta-analysis, a practice called 'best evidence synthesis'.[73] Other meta-analysts would include weaker studies, and add a study-level predictor variable that reflects the methodological quality of the studies to examine the effect of study quality on the effect size.[74] However, others have argued that a better approach is to preserve information about the variance in the study sample, casting as wide a net as possible, and that methodological selection criteria introduce unwanted subjectivity, defeating the purpose of the approach.[75]
Publication bias: the file drawer problem

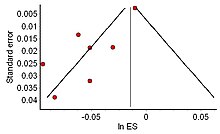
Another potential pitfall is the reliance on the available body of published studies, which may create exaggerated outcomes due to publication bias, as studies which show negative results or insignificant results are less likely to be published. For example, pharmaceutical companies have been known to hide negative studies and researchers may have overlooked unpublished studies such as dissertation studies or conference abstracts that did not reach publication. This is not easily solved, as one cannot know how many studies have gone unreported.[76]
This file drawer problem (characterized by negative or non-significant results being tucked away in a cabinet), can result in a biased distribution of effect sizes thus creating a serious base rate fallacy, in which the significance of the published studies is overestimated, as other studies were either not submitted for publication or were rejected. This should be seriously considered when interpreting the outcomes of a meta-analysis.[76][5]
The distribution of effect sizes can be visualized with a funnel plot which (in its most common version) is a scatter plot of standard error versus the effect size. It makes use of the fact that the smaller studies (thus larger standard errors) have more scatter of the magnitude of effect (being less precise) while the larger studies have less scatter and form the tip of the funnel. If many negative studies were not published, the remaining positive studies give rise to a funnel plot in which the base is skewed to one side (asymmetry of the funnel plot). In contrast, when there is no publication bias, the effect of the smaller studies has no reason to be skewed to one side and so a symmetric funnel plot results. This also means that if no publication bias is present, there would be no relationship between standard error and effect size.[77] A negative or positive relation between standard error and effect size would imply that smaller studies that found effects in one direction only were more likely to be published and/or to be submitted for publication.
Apart from the visual funnel plot, statistical methods for detecting publication bias have also been proposed. These are controversial because they typically have low power for detection of bias, but also may make false positives under some circumstances.[78] For instance small study effects (biased smaller studies), wherein methodological differences between smaller and larger studies exist, may cause asymmetry in effect sizes that resembles publication bias. However, small study effects may be just as problematic for the interpretation of meta-analyses, and the imperative is on meta-analytic authors to investigate potential sources of bias.
A Tandem Method for analyzing publication bias has been suggested for cutting down false positive error problems.[79] This Tandem method consists of three stages. Firstly, one calculates Orwin's fail-safe N, to check how many studies should be added in order to reduce the test statistic to a trivial size. If this number of studies is larger than the number of studies used in the meta-analysis, it is a sign that there is no publication bias, as in that case, one needs a lot of studies to reduce the effect size. Secondly, one can do an Egger's regression test, which tests whether the funnel plot is symmetrical. As mentioned before: a symmetrical funnel plot is a sign that there is no publication bias, as the effect size and sample size are not dependent. Thirdly, one can do the trim-and-fill method, which imputes data if the funnel plot is asymmetrical.
The problem of publication bias is not trivial as it is suggested that 25% of meta-analyses in the psychological sciences may have suffered from publication bias.[79] However, low power of existing tests and problems with the visual appearance of the funnel plot remain an issue, and estimates of publication bias may remain lower than what truly exists.
Most discussions of publication bias focus on journal practices favoring publication of statistically significant findings. However, questionable research practices, such as reworking statistical models until significance is achieved, may also favor statistically significant findings in support of researchers' hypotheses.[80][81]
Studies often do not report the effects when they do not reach statistical significance[citation needed]. For example, they may simply say that the groups did not show statistically significant differences, without reporting any other information (e.g. a statistic or p-value). Exclusion of these studies would lead to a situation similar to publication bias, but their inclusion (assuming null effects) would also bias the meta-analysis. MetaNSUE, a method created by Joaquim Radua, has shown to allow researchers to include unbiasedly these studies.[82] Its steps are as follows:
- Maximum likelihood estimation of the meta-analytic effect and the heterogeneity between studies.
- Multiple imputation of the NSUEs adding noise to the estimate of the effect.
- Separate meta-analyses for each imputed dataset.
- Pooling of the results of these meta-analyses.
Other weaknesses are that it has not been determined if the statistically most accurate method for combining results is the fixed, IVhet, random or quality effect models, though the criticism against the random effects model is mounting because of the perception that the new random effects (used in meta-analysis) are essentially formal devices to facilitate smoothing or shrinkage and prediction may be impossible or ill-advised.[83] The main problem with the random effects approach is that it uses the classic statistical thought of generating a "compromise estimator" that makes the weights close to the naturally weighted estimator if heterogeneity across studies is large but close to the inverse variance weighted estimator if the between study heterogeneity is small. However, what has been ignored is the distinction between the model we choose to analyze a given dataset, and the mechanism by which the data came into being.[84] A random effect can be present in either of these roles, but the two roles are quite distinct. There's no reason to think the analysis model and data-generation mechanism (model) are similar in form, but many sub-fields of statistics have developed the habit of assuming, for theory and simulations, that the data-generation mechanism (model) is identical to the analysis model we choose (or would like others to choose). As a hypothesized mechanisms for producing the data, the random effect model for meta-analysis is silly and it is more appropriate to think of this model as a superficial description and something we choose as an analytical tool – but this choice for meta-analysis may not work because the study effects are a fixed feature of the respective meta-analysis and the probability distribution is only a descriptive tool.[84]
Problems arising from agenda-driven bias
The most severe fault in meta-analysis[85] often occurs when the person or persons doing the meta-analysis have an economic, social, or political agenda such as the passage or defeat of legislation. People with these types of agendas may be more likely to abuse meta-analysis due to personal bias. For example, researchers favorable to the author's agenda are likely to have their studies cherry-picked while those not favorable will be ignored or labeled as "not credible". In addition, the favored authors may themselves be biased or paid to produce results that support their overall political, social, or economic goals in ways such as selecting small favorable data sets and not incorporating larger unfavorable data sets. The influence of such biases on the results of a meta-analysis is possible because the methodology of meta-analysis is highly malleable.[9]
A 2011 study done to disclose possible conflicts of interests in underlying research studies used for medical meta-analyses reviewed 29 meta-analyses and found that conflicts of interests in the studies underlying the meta-analyses were rarely disclosed. The 29 meta-analyses included 11 from general medicine journals, 15 from specialty medicine journals, and three from the Cochrane Database of Systematic Reviews. The 29 meta-analyses reviewed a total of 509 randomized controlled trials (RCTs). Of these, 318 RCTs reported funding sources, with 219 (69%) receiving funding from industry (i.e. one or more authors having financial ties to the pharmaceutical industry). Of the 509 RCTs, 132 reported author conflict of interest disclosures, with 91 studies (69%) disclosing one or more authors having financial ties to industry. The information was, however, seldom reflected in the meta-analyses. Only two (7%) reported RCT funding sources and none reported RCT author-industry ties. The authors concluded "without acknowledgment of COI due to industry funding or author industry financial ties from RCTs included in meta-analyses, readers' understanding and appraisal of the evidence from the meta-analysis may be compromised."[86]
For example, in 1998, a US federal judge found that the United States Environmental Protection Agency had abused the meta-analysis process to produce a study claiming cancer risks to non-smokers from environmental tobacco smoke (ETS) with the intent to influence policy makers to pass smoke-free–workplace laws. The judge found that:
EPA's study selection is disturbing. First, there is evidence in the record supporting the accusation that EPA "cherry picked" its data. Without criteria for pooling studies into a meta-analysis, the court cannot determine whether the exclusion of studies likely to disprove EPA's a priori hypothesis was coincidence or intentional. Second, EPA's excluding nearly half of the available studies directly conflicts with EPA's purported purpose for analyzing the epidemiological studies and conflicts with EPA's Risk Assessment Guidelines. See ETS Risk Assessment at 4-29 ("These data should also be examined in the interest of weighing all the available evidence, as recommended by EPA's carcinogen risk assessment guidelines (U.S. EPA, 1986a) (emphasis added)). Third, EPA's selective use of data conflicts with the Radon Research Act. The Act states EPA's program shall "gather data and information on all aspects of indoor air quality" (Radon Research Act § 403(a)(1)) (emphasis added).[87]
As a result of the abuse, the court vacated Chapters 1–6 of and the Appendices to EPA's "Respiratory Health Effects of Passive Smoking: Lung Cancer and other Disorders".[87]
Weak inclusion standards lead to misleading conclusions
Meta-analyses in education are often not restrictive enough in regards to the methodological quality of the studies they include. For example, studies that include small samples or researcher-made measures lead to inflated effect size estimates.[88] However, this problem also troubles meta-analysis of clinical trials. The use of different quality assessment tools (QATs) lead to including different studies and obtaining conflicting estimates of average treatment effects[89][90].
Applications in modern science
Modern statistical meta-analysis does more than just combine the effect sizes of a set of studies using a weighted average. It can test if the outcomes of studies show more variation than the variation that is expected because of the sampling of different numbers of research participants. Additionally, study characteristics such as measurement instrument used, population sampled, or aspects of the studies' design can be coded and used to reduce variance of the estimator (see statistical models above). Thus some methodological weaknesses in studies can be corrected statistically. Other uses of meta-analytic methods include the development and validation of clinical prediction models, where meta-analysis may be used to combine individual participant data from different research centers and to assess the model's generalisability,[91][92] or even to aggregate existing prediction models.[93]
Meta-analysis can be done with single-subject design as well as group research designs. This is important because much research has been done with single-subject research designs. Considerable dispute exists for the most appropriate meta-analytic technique for single subject research.[94]
Meta-analysis leads to a shift of emphasis from single studies to multiple studies. It emphasizes the practical importance of the effect size instead of the statistical significance of individual studies. This shift in thinking has been termed "meta-analytic thinking". The results of a meta-analysis are often shown in a forest plot.
Results from studies are combined using different approaches. One approach frequently used in meta-analysis in health care research is termed 'inverse variance method'. The average effect size across all studies is computed as a weighted mean, whereby the weights are equal to the inverse variance of each study's effect estimator. Larger studies and studies with less random variation are given greater weight than smaller studies. Other common approaches include the Mantel–Haenszel method[95] and the Peto method.[96]
Seed-based d mapping (formerly signed differential mapping, SDM) is a statistical technique for meta-analyzing studies on differences in brain activity or structure which used neuroimaging techniques such as fMRI, VBM or PET.
Different high throughput techniques such as microarrays have been used to understand Gene expression. MicroRNA expression profiles have been used to identify differentially expressed microRNAs in particular cell or tissue type or disease conditions or to check the effect of a treatment. A meta-analysis of such expression profiles was performed to derive novel conclusions and to validate the known findings.[97]
See also
- Estimation statistics
- Metascience
- Newcastle–Ottawa scale
- Reporting bias
- Review journal
- Secondary research
- Study heterogeneity
- Systematic review
- Galbraith plot
- Data aggregation
References
- ^ "Oxford Centre for Evidence-Based Medicine: Levels of Evidence (March 2009) — Centre for Evidence-Based Medicine (CEBM), University of Oxford". www.cebm.ox.ac.uk. Retrieved 21 December 2021.
- ^ National Institute for Health and Care Excellence Developing NICE guidelines: The manual National Institute for Health and Care Excellence, London (2014) Retrieved from: https://www.nice.org.uk/media/default/about/what-we-do/our-programmes/developing-nice-guidelines-the-manual.pdf Access, Accessed 21th Dec 2020
- ^ Greenland S, O' Rourke K: Meta-Analysis. Page 652 in Modern Epidemiology, 3rd ed. Edited by Rothman KJ, Greenland S, Lash T. Lippincott Williams and Wilkins; 2008.
- ^ Tabery J (9 May 2014). Beyond Versus: The Struggle to Understand the Interaction of Nature and Nurture. MIT Press. ISBN 978-0-262-02737-3.
- ^ a b Hunter JE, Schmidt FL (1990). Methods of Meta-Analysis: Correcting Error and Bias in Research Findings. Newbury Park, California; London; New Delhi: SAGE Publications.
- ^ Maziarz M (December 2021). "Is meta-analysis of RCTs assessing the efficacy of interventions a reliable source of evidence for therapeutic decisions?". Studies in History and Philosophy of Science. 91: 159–167. doi:10.1016/j.shpsa.2021.11.007. PMID 34922183. S2CID 245241150.
- ^ Feinstein AR (January 1995). "Meta-analysis: statistical alchemy for the 21st century". Journal of Clinical Epidemiology. The Potsdam International Consultation on Meta-Analysis. 48 (1): 71–79. doi:10.1016/0895-4356(94)00110-C. PMID 7853050.
- ^ Walker E, Hernandez AV, Kattan MW (June 2008). "Meta-analysis: Its strengths and limitations". Cleveland Clinic Journal of Medicine. 75 (6): 431–439. doi:10.3949/ccjm.75.6.431. PMID 18595551.
- ^ a b Stegenga J (December 2011). "Is meta-analysis the platinum standard of evidence?". Studies in History and Philosophy of Biological and Biomedical Sciences. 42 (4): 497–507. doi:10.1016/j.shpsc.2011.07.003. PMID 22035723.
- ^ Guolo A, Varin C (June 2017). "Random-effects meta-analysis: the number of studies matters". Statistical Methods in Medical Research. 26 (3): 1500–1518. doi:10.1177/0962280215583568. PMID 25953957. S2CID 24423336.
- ^ Wanous JP, Sullivan SE, Malinak J (1989). "The role of judgment calls in meta-analysis". Journal of Applied Psychology. 74 (2): 259–264. doi:10.1037/0021-9010.74.2.259. ISSN 0021-9010.
- ^ "Glossary at Cochrane Collaboration". cochrane.org.
- ^ Gravetter FJ, Forzano LB (1 January 2018). Research Methods for the Behavioral Sciences. Cengage Learning. p. 36. ISBN 9781337613316.
Some examples of secondary sources are (1) books and textbooks in which the author describes and summarizes past research, (2) review articles or meta-analyses...
- ^ Adams KA, Lawrence EK (2 February 2018). Research Methods, Statistics, and Applications. SAGE Publications. ISBN 9781506350462.
The most common types of secondary sources found in academic journals are literature reviews and meta-analyses.
- ^ Rühlemann MC, Hermes BM, Bang C, Doms S, Moitinho-Silva L, Thingholm LB, et al. (February 2021). "Genome-wide association study in 8,956 German individuals identifies influence of ABO histo-blood groups on gut microbiome". Nature Genetics. 53 (2): 147–155. doi:10.1038/s41588-020-00747-1. PMID 33462482. S2CID 231641761.
- ^ Plackett RL (1958). "Studies in the History of Probability and Statistics: Vii. The Principle of the Arithmetic Mean". Biometrika. 45 (1–2): 133. doi:10.1093/biomet/45.1-2.130.
- ^ Pearson K (November 1904). "Report on Certain Enteric Fever Inoculation Statistics". British Medical Journal. 2 (2288): 1243–1246. doi:10.1136/bmj.2.2288.1243. PMC 2355479. PMID 20761760.
- ^ Nordmann AJ, Kasenda B, Briel M (9 March 2012). "Meta-analyses: what they can and cannot do". Swiss Medical Weekly. 142: w13518. doi:10.4414/smw.2012.13518. PMID 22407741.
- ^ O'Rourke K (December 2007). "An historical perspective on meta-analysis: dealing quantitatively with varying study results". Journal of the Royal Society of Medicine. 100 (12): 579–582. doi:10.1258/jrsm.100.12.579. PMC 2121629. PMID 18065712.
- ^ Pratt JG, Rhine JB, Smith BM, Stuart CE, Greenwood JA. Extra-Sensory Perception after Sixty Years: A Critical Appraisal of the Research in Extra-Sensory Perception. New York: Henry Holt, 1940
- ^ Glass GV (1976). "Primary, secondary, and meta-analysis of research". Educational Researcher. 5 (10): 3–8. doi:10.3102/0013189X005010003. S2CID 3185455.
- ^ Cochran WG (1937). "Problems Arising in the Analysis of a Series of Similar Experiments". Journal of the Royal Statistical Society. 4 (1): 102–118. doi:10.2307/2984123. JSTOR 2984123.
- ^ Cochran WG, Carroll SP (1953). "A Sampling Investigation of the Efficiency of Weighting Inversely as the Estimated Variance". Biometrics. 9 (4): 447–459. doi:10.2307/3001436. JSTOR 3001436.
- ^ Gurevitch J, Koricheva J, Nakagawa S, Stewart G (March 2018). "Meta-analysis and the science of research synthesis". Nature. 555 (7695): 175–182. Bibcode:2018Natur.555..175G. doi:10.1038/nature25753. PMID 29517004. S2CID 3761687.
- ^ Gurevitch J, Morrow LL, Wallace A, Walsh JS (1992). "A Meta-Analysis of Competition in Field Experiments". The American Naturalist. 140 (4): 539–572. doi:10.1086/285428. ISSN 0003-0147. JSTOR 2462913. S2CID 205982939.
- ^ Gurevitch K (7 March 2018). "Forty years of meta-analysis: We need evidence-based answers more than ever". Research Data at Springer Nature. Retrieved 24 July 2021.
- ^ "The PRISMA statement". Prisma-statement.org. 2 February 2012. Retrieved 2 February 2012.
- ^ Debray TP, Moons KG, van Valkenhoef G, Efthimiou O, Hummel N, Groenwold RH, Reitsma JB (December 2015). "Get real in individual participant data (IPD) meta-analysis: a review of the methodology". Research Synthesis Methods. 6 (4): 293–309. doi:10.1002/jrsm.1160. PMC 5042043. PMID 26287812.
- ^ Debray TP, Moons KG, Abo-Zaid GM, Koffijberg H, Riley RD (2013). "Individual participant data meta-analysis for a binary outcome: one-stage or two-stage?". PloS One. 8 (4): e60650. Bibcode:2013PLoSO...860650D. doi:10.1371/journal.pone.0060650. PMC 3621872. PMID 23585842.
- ^ Burke DL, Ensor J, Riley RD (February 2017). "Meta-analysis using individual participant data: one-stage and two-stage approaches, and why they may differ". Statistics in Medicine. 36 (5): 855–875. doi:10.1002/sim.7141. PMC 5297998. PMID 27747915.
- ^ Helfenstein U (March 2002). "Data and models determine treatment proposals--an illustration from meta-analysis". Postgraduate Medical Journal. 78 (917): 131–134. doi:10.1136/pmj.78.917.131. PMC 1742301. PMID 11884693.
- ^ Senn S (March 2007). "Trying to be precise about vagueness". Statistics in Medicine. 26 (7): 1417–1430. doi:10.1002/sim.2639. PMID 16906552.
- ^ a b Al Khalaf MM, Thalib L, Doi SA (February 2011). "Combining heterogenous studies using the random-effects model is a mistake and leads to inconclusive meta-analyses". Journal of Clinical Epidemiology. 64 (2): 119–123. doi:10.1016/j.jclinepi.2010.01.009. PMID 20409685.
- ^ a b Brockwell SE, Gordon IR (March 2001). "A comparison of statistical methods for meta-analysis". Statistics in Medicine. 20 (6): 825–840. doi:10.1002/sim.650. PMID 11252006.
- ^ a b c Noma H (December 2011). "Confidence intervals for a random-effects meta-analysis based on Bartlett-type corrections". Statistics in Medicine. 30 (28): 3304–3312. doi:10.1002/sim.4350. hdl:2433/152046. PMID 21964669.
- ^ Brockwell SE, Gordon IR (November 2007). "A simple method for inference on an overall effect in meta-analysis". Statistics in Medicine. 26 (25): 4531–4543. doi:10.1002/sim.2883. PMID 17397112.
- ^ Sidik K, Jonkman JN (November 2002). "A simple confidence interval for meta-analysis". Statistics in Medicine. 21 (21): 3153–3159. doi:10.1002/sim.1262. PMID 12375296.
- ^ Jackson D, Bowden J (January 2009). "A re-evaluation of the 'quantile approximation method' for random effects meta-analysis". Statistics in Medicine. 28 (2): 338–348. doi:10.1002/sim.3487. PMC 2991773. PMID 19016302.
- ^ Poole C, Greenland S (September 1999). "Random-effects meta-analyses are not always conservative". American Journal of Epidemiology. 150 (5): 469–475. doi:10.1093/oxfordjournals.aje.a010035. PMID 10472946.
- ^ Riley RD, Higgins JP, Deeks JJ (February 2011). "Interpretation of random effects meta-analyses". BMJ. 342: d549. doi:10.1136/bmj.d549. PMID 21310794. S2CID 32994689.
- ^ Kriston L (March 2013). "Dealing with clinical heterogeneity in meta-analysis. Assumptions, methods, interpretation". International Journal of Methods in Psychiatric Research. 22 (1): 1–15. doi:10.1002/mpr.1377. PMC 6878481. PMID 23494781.
- ^ DerSimonian R, Laird N (September 1986). "Meta-analysis in clinical trials". Controlled Clinical Trials. 7 (3): 177–188. doi:10.1016/0197-2456(86)90046-2. PMID 3802833.
- ^ Kontopantelis E, Reeves D (1 August 2010). "Metaan: Random-effects meta-analysis". Stata Journal. 10 (3): 395–407. doi:10.1177/1536867X1001000307 – via ResearchGate.
- ^ Kontopantelis E, Reeves D (2009). "MetaEasy:A Meta-Analysis Add-In for Microsoft Excel, Journal of Statistical Software 2009". Journal of Statistical Software. 30 (7). doi:10.18637/jss.v030.i07.
- ^ "Developer's website". Statanalysis.co.uk. Retrieved 18 September 2018.
- ^ Kontopantelis E, Reeves D (August 2012). "Performance of statistical methods for meta-analysis when true study effects are non-normally distributed: A simulation study". Statistical Methods in Medical Research. 21 (4): 409–426. doi:10.1177/0962280210392008. PMID 21148194. S2CID 152379.
- ^ Kontopantelis E, Reeves D (December 2012). "Performance of statistical methods for meta-analysis when true study effects are non-normally distributed: a comparison between DerSimonian-Laird and restricted maximum likelihood". Statistical Methods in Medical Research. 21 (6): 657–659. doi:10.1177/0962280211413451. PMID 23171971. S2CID 44928009.
- ^ Kontopantelis E, Springate DA, Reeves D (2013). Friede T (ed.). "A re-analysis of the Cochrane Library data: the dangers of unobserved heterogeneity in meta-analyses". PloS One. 8 (7): e69930. Bibcode:2013PLoSO...869930K. doi:10.1371/journal.pone.0069930. PMC 3724681. PMID 23922860.
- ^ Kontopantelis E, Reeves D (27 September 2013). "A short guide and a forest plot command (ipdforest) for one-stage meta-analysis". Stata Journal. 13 (3): 574–587. doi:10.1177/1536867X1301300308 – via ResearchGate.
- ^ a b c "MetaXL User Guide" (PDF). Retrieved 18 September 2018.
- ^ a b c d "MetaXL software page". Epigear.com. 3 June 2017. Retrieved 18 September 2018.
- ^ Doi SA, Barendregt JJ, Khan S, Thalib L, Williams GM (November 2015). "Advances in the meta-analysis of heterogeneous clinical trials I: The inverse variance heterogeneity model". Contemporary Clinical Trials. 45 (Pt A): 130–138. doi:10.1016/j.cct.2015.05.009. hdl:1885/17083. PMID 26003435.
- ^ a b Doi SA, Thalib L (January 2008). "A quality-effects model for meta-analysis". Epidemiology. 19 (1): 94–100. doi:10.1097/EDE.0b013e31815c24e7. PMID 18090860. S2CID 29723291.
- ^ Doi SA, Barendregt JJ, Mozurkewich EL (March 2011). "Meta-analysis of heterogeneous clinical trials: an empirical example". Contemporary Clinical Trials. 32 (2): 288–298. doi:10.1016/j.cct.2010.12.006. PMID 21147265.
- ^ Doi SA, Barendregt JJ, Khan S, Thalib L, Williams GM (July 2015). "Simulation Comparison of the Quality Effects and Random Effects Methods of Meta-analysis". Epidemiology. 26 (4): e42–e44. doi:10.1097/EDE.0000000000000289. PMID 25872162.
- ^ Doi SA, Barendregt JJ, Khan S, Thalib L, Williams GM (November 2015). "Advances in the meta-analysis of heterogeneous clinical trials II: The quality effects model". Contemporary Clinical Trials. 45 (Pt A): 123–129. doi:10.1016/j.cct.2015.05.010. PMID 26003432.
- ^ Bucher HC, Guyatt GH, Griffith LE, Walter SD (June 1997). "The results of direct and indirect treatment comparisons in meta-analysis of randomized controlled trials". Journal of Clinical Epidemiology. 50 (6): 683–691. doi:10.1016/s0895-4356(97)00049-8. PMID 9250266.
- ^ a b van Valkenhoef G, Lu G, de Brock B, Hillege H, Ades AE, Welton NJ (December 2012). "Automating network meta-analysis". Research Synthesis Methods. 3 (4): 285–299. doi:10.1002/jrsm.1054. PMID 26053422.
- ^ Brooks SP, Gelman A (1998). "General methods for monitoring convergence of iterative simulations" (PDF). Journal of Computational and Graphical Statistics. 7 (4): 434–455. doi:10.1080/10618600.1998.10474787.
- ^ van Valkenhoef G, Lu G, de Brock B, Hillege H, Ades AE, Welton NJ. Automating network meta-analysis. Res Synth Methods. 2012 Dec;3(4):285-99.
- ^ a b Senn S, Gavini F, Magrez D, Scheen A (April 2013). "Issues in performing a network meta-analysis". Statistical Methods in Medical Research. 22 (2): 169–189. doi:10.1177/0962280211432220. PMID 22218368. S2CID 10860031.
- ^ White IR (2011). "Multivariate random-effects meta-regression: updates to mvmeta". The Stata Journal. 11 (2): 255–270. doi:10.1177/1536867X1101100206.
- ^ van Valkenhoef G, Lu G, de Brock B, Hillege H, Ades AE, Welton NJ. Automating network meta-analysis. Res Synth Methods. 2012 Dec;3(4):285-99
- ^ Willis BH, Hyde CJ (May 2014). "Estimating a test's accuracy using tailored meta-analysis-How setting-specific data may aid study selection". Journal of Clinical Epidemiology. 67 (5): 538–546. doi:10.1016/j.jclinepi.2013.10.016. PMID 24447592.
- ^ Willis BH, Hyde CJ (August 2015). "What is the test's accuracy in my practice population? Tailored meta-analysis provides a plausible estimate". Journal of Clinical Epidemiology. 68 (8): 847–854. doi:10.1016/j.jclinepi.2014.10.002. PMID 25479685.
- ^ Zhang H, Deng L, Schiffman M, Qin J, Yu K (2020). "Generalized integration model for improved statistical inference by leveraging external summary data". Biometrika. 107 (3): 689–703. doi:10.1093/biomet/asaa014.
- ^ Higgins JP, Altman DG, Gøtzsche PC, Jüni P, Moher D, Oxman AD, et al. (October 2011). "The Cochrane Collaboration's tool for assessing risk of bias in randomised trials". BMJ. 343: d5928. doi:10.1136/bmj.d5928. PMC 3196245. PMID 22008217.
- ^ Whiting PF, Rutjes AW, Westwood ME, Mallett S, Deeks JJ, Reitsma JB, et al. (October 2011). "QUADAS-2: a revised tool for the quality assessment of diagnostic accuracy studies". Annals of Internal Medicine. 155 (8): 529–536. doi:10.7326/0003-4819-155-8-201110180-00009. PMID 22007046.
- ^ Royston P, Parmar MK, Sylvester R (March 2004). "Construction and validation of a prognostic model across several studies, with an application in superficial bladder cancer". Statistics in Medicine. 23 (6): 907–926. doi:10.1002/sim.1691. PMID 15027080.
- ^ Willis BH, Riley RD (September 2017). "Measuring the statistical validity of summary meta-analysis and meta-regression results for use in clinical practice". Statistics in Medicine. 36 (21): 3283–3301. doi:10.1002/sim.7372. PMC 5575530. PMID 28620945.
- ^ Riley RD, Ahmed I, Debray TP, Willis BH, Noordzij JP, Higgins JP, Deeks JJ (June 2015). "Summarising and validating test accuracy results across multiple studies for use in clinical practice". Statistics in Medicine. 34 (13): 2081–2103. doi:10.1002/sim.6471. PMC 4973708. PMID 25800943.
- ^ LeLorier J, Grégoire G, Benhaddad A, Lapierre J, Derderian F (August 1997). "Discrepancies between meta-analyses and subsequent large randomized, controlled trials". The New England Journal of Medicine. 337 (8): 536–542. doi:10.1056/NEJM199708213370806. PMID 9262498.
- ^ a b Slavin RE (1986). "Best-Evidence Synthesis: An Alternative to Meta-Analytic and Traditional Reviews". Educational Researcher. 15 (9): 5–9. doi:10.3102/0013189X015009005. S2CID 146457142.
- ^ Hunter JE, Schmidt FL, Jackson GB, et al. (American Psychological Association. Division of Industrial-Organizational Psychology) (1982). Meta-analysis: cumulating research findings across studies. Beverly Hills, California: Sage. ISBN 978-0-8039-1864-1.
- ^ Glass GV, McGaw B, Smith ML (1981). Meta-analysis in social research. Beverly Hills, California: Sage Publications. ISBN 978-0-8039-1633-3.
- ^ a b Rosenthal R (1979). "The "File Drawer Problem" and the Tolerance for Null Results". Psychological Bulletin. 86 (3): 638–641. doi:10.1037/0033-2909.86.3.638. S2CID 36070395.
- ^ Light RJ, Pillemer DB (1984). Summing up : the science of reviewing research. Cambridge, Massachusetts: Harvard University Press. ISBN 978-0-674-85431-4.
- ^ Ioannidis JP, Trikalinos TA (April 2007). "The appropriateness of asymmetry tests for publication bias in meta-analyses: a large survey". Cmaj. 176 (8): 1091–1096. doi:10.1503/cmaj.060410. PMC 1839799. PMID 17420491.
- ^ a b Ferguson CJ, Brannick MT (March 2012). "Publication bias in psychological science: prevalence, methods for identifying and controlling, and implications for the use of meta-analyses". Psychological Methods. 17 (1): 120–128. doi:10.1037/a0024445. PMID 21787082.
- ^ Simmons JP, Nelson LD, Simonsohn U (November 2011). "False-positive psychology: undisclosed flexibility in data collection and analysis allows presenting anything as significant". Psychological Science. 22 (11): 1359–1366. doi:10.1177/0956797611417632. PMID 22006061.
- ^ LeBel, E.; Peters, K. (2011). "Fearing the future of empirical psychology: Bem's (2011) evidence of psi as a case study of deficiencies in modal research practice" (PDF). Review of General Psychology. 15 (4): 371–379. doi:10.1037/a0025172. S2CID 51686730. Archived from the original (PDF) on 24 November 2012.
- ^ Radua J, Schmidt A, Borgwardt S, Heinz A, Schlagenhauf F, McGuire P, Fusar-Poli P (December 2015). "Ventral Striatal Activation During Reward Processing in Psychosis: A Neurofunctional Meta-Analysis". JAMA Psychiatry. 72 (12): 1243–1251. doi:10.1001/jamapsychiatry.2015.2196. PMID 26558708.
- ^ Hodges, Jim, and Clayton, Murray K. Random Effects: Old and New. Statistical Science XX: XX–XX. URL http://www Archived 24 May 2011 at the Wayback Machine. biostat. umn. edu/~ hodges/Hodges-ClaytonREONsubToStatSci (2011)
- ^ a b Hodges JS. Random effects old and new. In Hodges JS. Richly parameterized linear models: additive, time series, and spatial models using random effects. USA: CRC Press, 2013: 285–302.
- ^ H. Sabhan
- ^ Roseman M, Milette K, Bero LA, Coyne JC, Lexchin J, Turner EH, Thombs BD (2011), "Reporting of Conflicts of Interest in Meta-analyses of Trials of Pharmacological Treatments", Journal of the American Medical Association, 305 (10): 1008–1017, doi:10.1001/jama.2011.257, hdl:11370/d4a95ee2-429f-45a4-a917-d794ee954797, PMID 21386079
- ^ a b "The Osteen Decision". The United States District Court for the Middle District of North Carolina. 17 July 1998. Retrieved 18 March 2017.
- ^ Cheung AC, Slavin RE (1 June 2016). "How Methodological Features Affect Effect Sizes in Education". Educational Researcher. 45 (5): 283–292. doi:10.3102/0013189X16656615. ISSN 0013-189X. S2CID 148531062.
- ^ Jüni P, Witschi A, Bloch R, Egger M (September 1999). "The hazards of scoring the quality of clinical trials for meta-analysis". Jama. 282 (11): 1054–1060. doi:10.1001/jama.282.11.1054. PMID 10493204.
- ^ Armijo-Olivo S, Fuentes J, Ospina M, Saltaji H, Hartling L (September 2013). "Inconsistency in the items included in tools used in general health research and physical therapy to evaluate the methodological quality of randomized controlled trials: a descriptive analysis". BMC Medical Research Methodology. 13 (1): 116. doi:10.1186/1471-2288-13-116. PMC 3848693. PMID 24044807.
{{cite journal}}
: CS1 maint: unflagged free DOI (link) - ^ Debray TP, Riley RD, Rovers MM, Reitsma JB, Moons KG (October 2015). "Individual participant data (IPD) meta-analyses of diagnostic and prognostic modeling studies: guidance on their use". PLoS Medicine. 12 (10): e1001886. doi:10.1371/journal.pmed.1001886. PMC 4603958. PMID 26461078.
{{cite journal}}
: CS1 maint: unflagged free DOI (link) - ^ Debray TP, Moons KG, Ahmed I, Koffijberg H, Riley RD (August 2013). "A framework for developing, implementing, and evaluating clinical prediction models in an individual participant data meta-analysis". Statistics in Medicine. 32 (18): 3158–3180. doi:10.1002/sim.5732. PMID 23307585.
- ^ Debray TP, Koffijberg H, Vergouwe Y, Moons KG, Steyerberg EW (October 2012). "Aggregating published prediction models with individual participant data: a comparison of different approaches". Statistics in Medicine. 31 (23): 2697–2712. doi:10.1002/sim.5412. PMID 22733546.
- ^ Van den Noortgate W, Onghena P (2007). "Aggregating Single-Case Results". The Behavior Analyst Today. 8 (2): 196–209. doi:10.1037/h0100613.
- ^ Mantel N, Haenszel W (April 1959). "Statistical aspects of the analysis of data from retrospective studies of disease". Journal of the National Cancer Institute. 22 (4): 719–748. doi:10.1093/jnci/22.4.719. PMID 13655060. S2CID 17698270.
- ^ "9.4.4.2 Peto odds ratio method". Cochrane Handbook for Systematic Reviews of Interventions v 5.1.0. March 2011.
- ^ Bargaje R, Hariharan M, Scaria V, Pillai B (January 2010). "Consensus miRNA expression profiles derived from interplatform normalization of microarray data". Rna. 16 (1): 16–25. doi:10.1261/rna.1688110. PMC 2802026. PMID 19948767.
Further reading
- Cooper H, Hedges LV, eds. (1994). The Handbook of Research Synthesis. New York: Russell Sage Foundation. ISBN 978-0-87154-226-7.
- Cornell JE, Mulrow CD (1999). "Meta-analysis". In Mellenbergh GJ (ed.). Research methodology in the life, behavioural, and social sciences. London: SAGE. pp. 285–323. ISBN 978-0-7619-5883-3.
- Normand SL (February 1999). "Meta-analysis: formulating, evaluating, combining, and reporting". Statistics in Medicine. 18 (3): 321–359. doi:10.1002/(SICI)1097-0258(19990215)18:3<321::AID-SIM28>3.0.CO;2-P. PMID 10070677.
- Sutton, A.J., Jones, D.R., Abrams, K.R., Sheldon, T.A., & Song, F. (2000). Methods for Meta-analysis in Medical Research. London: John Wiley. ISBN 0-471-49066-0
- Higgins JPT, Green S (editors). Cochrane Handbook for Systematic Reviews of Interventions Version 5.0.1 [updated September 2008]. The Cochrane Collaboration, 2008. Available from www.cochrane-handbook.org
- Thompson SG, Pocock SJ (November 1991). "Can meta-analyses be trusted?" (PDF). Lancet. 338 (8775): 1127–1130. doi:10.1016/0140-6736(91)91975-Z. PMID 1682553. S2CID 29743240. Archived from the original (PDF) on 22 November 2011. Retrieved 17 June 2011.. Explores two contrasting views: does meta-analysis provide "objective, quantitative methods for combining evidence from separate but similar studies" or merely "statistical tricks which make unjustified assumptions in producing oversimplified generalisations out of a complex of disparate studies"?
- Wilson, D. B., & Lipsey, M. W. (2001). Practical meta-analysis. Thousand Oaks: Sage publications. ISBN 0-7619-2168-0
- O'Rourke, K. (2007) Just the history from the combining of information: investigating and synthesizing what is possibly common in clinical observations or studies via likelihood. Oxford: University of Oxford, Department of Statistics. Gives technical background material and details on the "An historical perspective on meta-analysis" paper cited in the references.
- Owen, A. B. (2009). "Karl Pearson's meta-analysis revisited". Annals of Statistics, 37 (6B), 3867–3892. Supplementary report.
- Ellis, Paul D. (2010). The Essential Guide to Effect Sizes: An Introduction to Statistical Power, Meta-Analysis and the Interpretation of Research Results. United Kingdom: Cambridge University Press. ISBN 0-521-14246-6
- Bonett, DG (2020) Point-biserial correlation: Confidence intervals, hypothesis testing, meta-analysis, and sample size planning. British Journal of Mathematical and Statistical Psychology 73. doi: 10.1111/bmsp.12189
- Bonett DG, Price RM (September 2015). "Varying coefficient meta-analysis methods for odds ratios and risk ratios". Psychological Methods. 20 (3): 394–406. doi:10.1037/met0000032. PMID 25751513.
- Bonett DG, Price RM (November 2014). "Meta-analysis methods for risk differences". The British Journal of Mathematical and Statistical Psychology. 67 (3): 371–387. doi:10.1111/bmsp.12024. PMID 23962020.
- Bonett DG (December 2010). "Varying coefficient meta-analytic methods for alpha reliability". Psychological Methods. 15 (4): 368–385. doi:10.1037/a0020142. PMID 20853952. S2CID 207710319.
- Bonett DG (September 2009). "Meta-analytic interval estimation for standardized and unstandardized mean differences". Psychological Methods. 14 (3): 225–238. doi:10.1037/a0016619. PMID 19719359.
- Bonett DG (September 2008). "Meta-analytic interval estimation for bivariate correlations". Psychological Methods. 13 (3): 173–181. doi:10.1037/a0012868. PMID 18778150. S2CID 5690835.
External links

- Cochrane Handbook for Systematic Reviews of Interventions
- Meta-Analysis at 25 (Gene V Glass)
- Preferred Reporting Items for Systematic Reviews and Meta-Analyses (PRISMA) Statement, "an evidence-based minimum set of items for reporting in systematic reviews and meta-analyses."
- “metansue” R package and graphical interface
- Best Evidence Encyclopedia